Smart city network flow prediction method and system
A technology of network traffic and prediction method, which is applied in transmission systems, digital transmission systems, data exchange networks, etc., can solve problems such as obvious noise, inability to effectively capture the nonlinear characteristics of time series, unstable network traffic, etc., and achieve the goal of reducing interference Effect
- Summary
- Abstract
- Description
- Claims
- Application Information
AI Technical Summary
Problems solved by technology
Method used
Image
Examples
Embodiment Construction
[0048] In order to make the purpose, technical solutions and advantages of the present invention clearer, the technical solutions in the present invention will be clearly and completely described below in conjunction with the accompanying drawings in the present invention. Obviously, the described embodiments are part of the embodiments of the present invention , but not all examples. Based on the embodiments of the present invention, all other embodiments obtained by persons of ordinary skill in the art without creative efforts fall within the protection scope of the present invention.
[0049] Nonlinear models are currently more accurate than traditional predictive models, but their ability to capture long-term correlations is lower.
[0050]In recent years, deep learning methods have become the mainstream method for time series forecasting. Recurrent Neural Networks (RNNs), have shown their strengths in sequence modeling tasks. As its typical example, LSTM network can not...
PUM
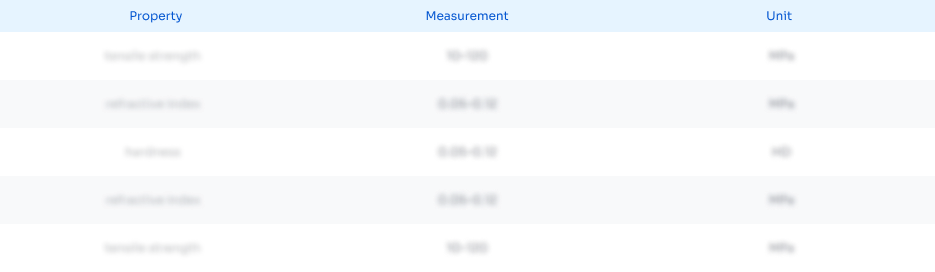
Abstract
Description
Claims
Application Information
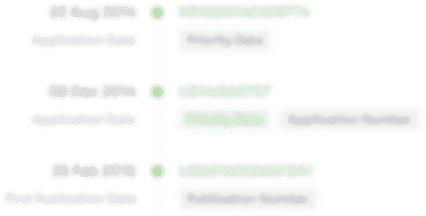
- R&D Engineer
- R&D Manager
- IP Professional
- Industry Leading Data Capabilities
- Powerful AI technology
- Patent DNA Extraction
Browse by: Latest US Patents, China's latest patents, Technical Efficacy Thesaurus, Application Domain, Technology Topic, Popular Technical Reports.
© 2024 PatSnap. All rights reserved.Legal|Privacy policy|Modern Slavery Act Transparency Statement|Sitemap|About US| Contact US: help@patsnap.com