A KPI abnormal early warning method for intelligent IT operation and maintenance system
An operation and maintenance system and abnormal technology, which is applied in the direction of network data indexing, instrumentation, and other database retrieval, can solve problems such as limiting the application of decision tree algorithms, and achieve the effect of reducing manual workload and ensuring accuracy
- Summary
- Abstract
- Description
- Claims
- Application Information
AI Technical Summary
Problems solved by technology
Method used
Image
Examples
Embodiment 1
[0067] Embodiment 1: as image 3 As shown, a KPI abnormal early warning method for an intelligent IT operation and maintenance system includes steps:
[0068] S1, determine the abnormal data of adjacent time, and form the abnormal data of adjacent time into an abnormal data group;
[0069] S2, determining the abnormal data transition process time group;
[0070] S3, calculating the trend fluctuation point within the initial time point of the abnormal data transition process time group in step S2;
[0071] S4, based on the data in steps S2 and S3, calculate and record trend information and maximum trend information;
[0072] S5, using the trend information and the maximum value trend information in step S4, to determine whether the real-time data is abnormal in the real-time abnormal warning.
[0073] Among the above five steps, step S1 to find historical abnormal data belongs to the data preparation stage. Steps S2, S3, and S4 perform feature engineering based on the histo...
Embodiment 2
[0075] Such as Figure 1~Figure 2 shown. On the basis of Embodiment 1, step S1 to determine the adjacent time abnormal data group refers to searching for data greater than or equal to the threshold from the historical KPI data, and forming adjacent abnormal data into an abnormal data group, including sub-steps:
[0076] S101, setting a threshold K, comparing the historical KPI data with the threshold K, marking the ones greater than or equal to K as 1, and marking the ones smaller than K as 0;
[0077] S102, traverse the historical KPI data, find the data whose current time point is 1, which is the abnormal data, and the previous time point is 0, which is the entry point time of the normal data, and record it in the time series time1_pre;
[0078] S103, traverse the historical KPI data, find out that the current time point is 1, which is the abnormal data, and the next time point is 0, which is the exit time of the normal data, and record it in the time series time1_last;
...
Embodiment 3
[0082] On the basis of Embodiment 2, step S2 determines the transition process time point of the abnormal data set based on the step S1 abnormal data set time1_pair, and the purpose of determining the transition process time point of the abnormal data set is for subsequent learning of the transition from the normal state to the abnormal state Prepare data for trend information, including sub-steps:
[0083] S201, traverse the abnormal entry and exit time pairs in the abnormal data group time1_pair in S105, push forward N time points for each abnormal entry point time, and record it in the time sequence time2_pre;
[0084] S202, traverse the abnormal entry and exit time pairs in the abnormal data group time1_pair, push back N time points for each abnormal exit point time, and record it in the time sequence time2_last;
[0085] S203, according to the time series obtained in S201 and S202, one-to-one correspondence is formed according to the index to form the abnormal data transi...
PUM
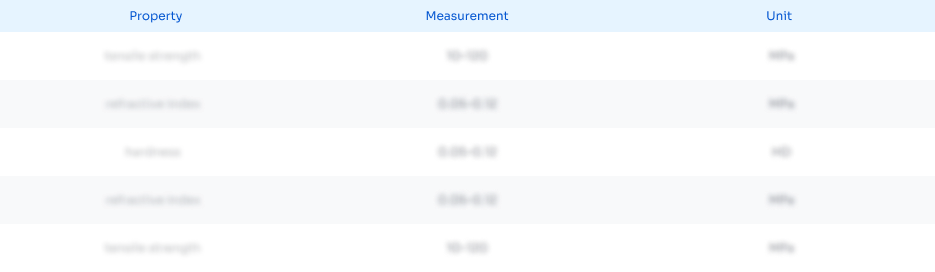
Abstract
Description
Claims
Application Information
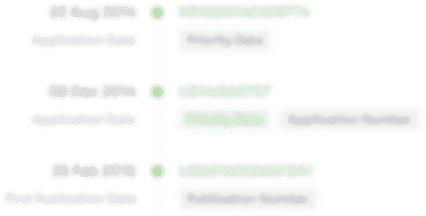
- R&D Engineer
- R&D Manager
- IP Professional
- Industry Leading Data Capabilities
- Powerful AI technology
- Patent DNA Extraction
Browse by: Latest US Patents, China's latest patents, Technical Efficacy Thesaurus, Application Domain, Technology Topic, Popular Technical Reports.
© 2024 PatSnap. All rights reserved.Legal|Privacy policy|Modern Slavery Act Transparency Statement|Sitemap|About US| Contact US: help@patsnap.com