Frequency spectrum prediction method based on radio frequency machine learning model driving
A machine learning model and spectrum prediction technology, applied in the field of communication, can solve the problems that the prediction performance cannot accurately reflect the real spectrum usage status, it is difficult to obtain a large amount of high-quality label data, and the convergence speed is slow, so as to improve the performance of spectrum prediction and overcome blackouts. Box effect, the effect of reducing the network scale
- Summary
- Abstract
- Description
- Claims
- Application Information
AI Technical Summary
Problems solved by technology
Method used
Image
Examples
Embodiment Construction
[0058] The present invention is described in further detail now in conjunction with accompanying drawing.
[0059] It should be noted that terms such as "upper", "lower", "left", "right", "front", and "rear" quoted in the invention are only for clarity of description, not for Limiting the practicable scope of the present invention, and changes or adjustments in their relative relationships, without substantial changes in the technical content, shall also be regarded as the practicable scope of the present invention.
[0060] figure 1 It is a flowchart of a spectrum prediction method driven by a radio frequency machine learning model according to an embodiment of the present invention. see figure 1 , the spectrum prediction method includes the following steps:
[0061] S1, collecting spectrum data, and performing preprocessing on the collected spectrum data.
[0062] S2. Determine the order of the autoregressive model according to the Akaike information criterion, and deter...
PUM
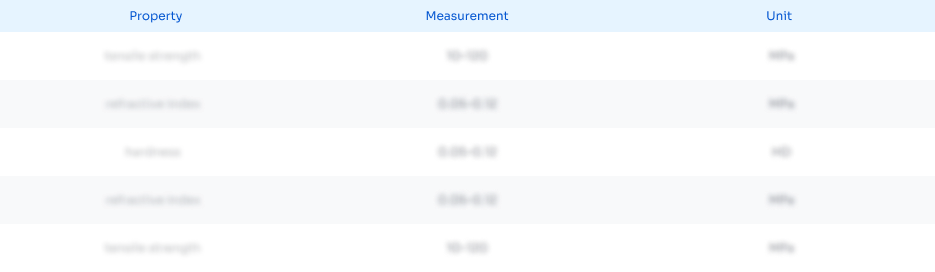
Abstract
Description
Claims
Application Information
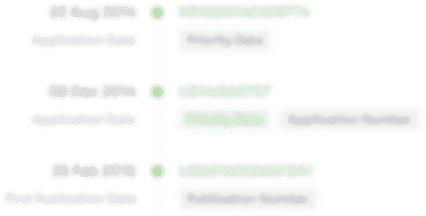
- R&D Engineer
- R&D Manager
- IP Professional
- Industry Leading Data Capabilities
- Powerful AI technology
- Patent DNA Extraction
Browse by: Latest US Patents, China's latest patents, Technical Efficacy Thesaurus, Application Domain, Technology Topic, Popular Technical Reports.
© 2024 PatSnap. All rights reserved.Legal|Privacy policy|Modern Slavery Act Transparency Statement|Sitemap|About US| Contact US: help@patsnap.com