A Surface Anomaly Detection Method Based on Hybrid Supervised Learning
An anomaly detection and supervised learning technology, applied in the field of surface anomaly detection based on hybrid supervised learning, can solve the problems of subjectivity and time-consuming, lack of training data, dependence, etc., to reduce the need for high-precision labeling, improve detection efficiency, and reduce labeling cost effect
- Summary
- Abstract
- Description
- Claims
- Application Information
AI Technical Summary
Problems solved by technology
Method used
Image
Examples
Embodiment Construction
[0041] In order to make those skilled in the art better understand the technical solutions of the present disclosure, the following will clearly and completely describe the technical solutions in the embodiments of the present disclosure with reference to the accompanying drawings in the embodiments of the present disclosure. Obviously, the described embodiments are only some, but not all, embodiments of the present disclosure. Based on the embodiments in the present disclosure, all other embodiments obtained by those of ordinary skill in the art without creative efforts shall fall within the protection scope of the present disclosure.
[0042] refer to Figure 1-Figure 7 As shown, the present invention provides a surface anomaly detection method based on hybrid supervised learning, and the method includes the following steps:
[0043] S1. Obtain image data including abnormal samples and normal samples and construct a corresponding data set, wherein abnormal samples are reco...
PUM
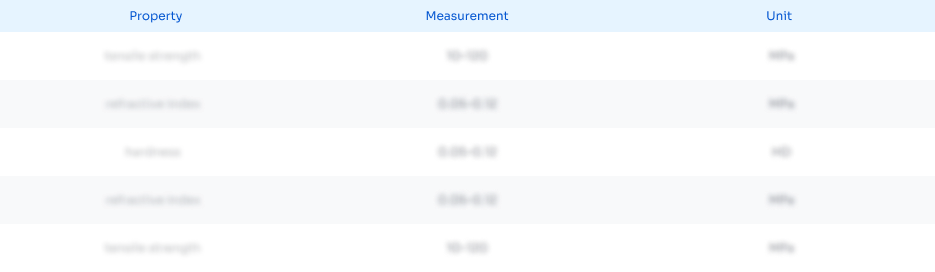
Abstract
Description
Claims
Application Information
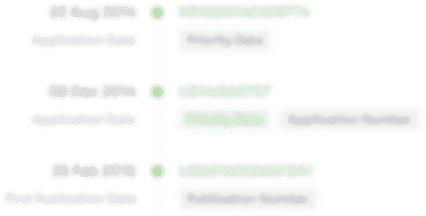
- R&D Engineer
- R&D Manager
- IP Professional
- Industry Leading Data Capabilities
- Powerful AI technology
- Patent DNA Extraction
Browse by: Latest US Patents, China's latest patents, Technical Efficacy Thesaurus, Application Domain, Technology Topic, Popular Technical Reports.
© 2024 PatSnap. All rights reserved.Legal|Privacy policy|Modern Slavery Act Transparency Statement|Sitemap|About US| Contact US: help@patsnap.com