Stomach cancer patient survival risk prediction method based on histopathology image and gene expression data
A gene expression and risk prediction technology, applied in medical images, neural learning methods, pathological reference, etc., can solve problems such as ignoring clinical information of patients, improve prediction effect and robustness, improve model prediction accuracy, and improve prediction ability Effect
- Summary
- Abstract
- Description
- Claims
- Application Information
AI Technical Summary
Problems solved by technology
Method used
Image
Examples
Embodiment
[0032] The present invention relates to a method for predicting the survival risk of gastric cancer patients based on histopathological images and gene expression data. The method aims to provide a fully automated deep convolutional neural network model to directly predict the survival risk of patients from gastric cancer histopathological images (DeepCox-SC model). Using the gastric cancer data in TCGA (The cancer genome atlas, cancer genome data set), it was found that the prognostic accuracy of DeepCox-SC exceeded the accuracy of manual annotation by pathologists. By further integrating histopathological images, clinical data and high-dimensional gene expression data to construct a DeepCox-SC multimodal fusion model, the prediction accuracy is further improved. The realization scheme of the inventive method specifically comprises the following contents:
[0033] 1. Data set preparation
[0034]Histopathological images, clinical data, and gene expression data of gastric ca...
PUM
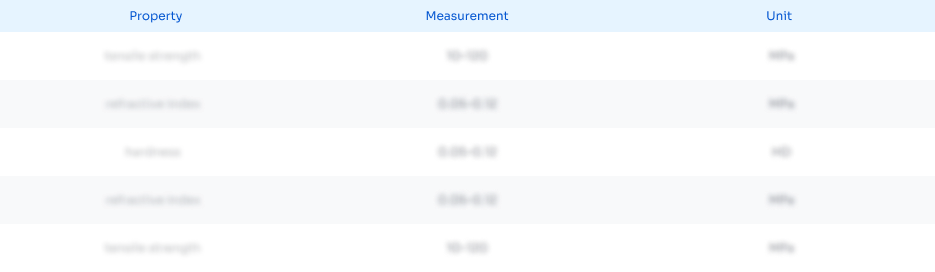
Abstract
Description
Claims
Application Information
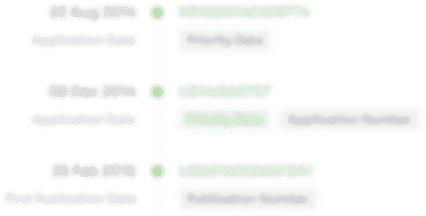
- R&D
- Intellectual Property
- Life Sciences
- Materials
- Tech Scout
- Unparalleled Data Quality
- Higher Quality Content
- 60% Fewer Hallucinations
Browse by: Latest US Patents, China's latest patents, Technical Efficacy Thesaurus, Application Domain, Technology Topic, Popular Technical Reports.
© 2025 PatSnap. All rights reserved.Legal|Privacy policy|Modern Slavery Act Transparency Statement|Sitemap|About US| Contact US: help@patsnap.com