Thermal error prediction model method for electric spindle with variable bearing pre-tightening force
A bearing pretightening force and prediction model technology, applied in the direction of nuclear method, calculation model, biological model, etc., can solve the problem of increasing calculation workload, new samples cannot predict the results well, and it is difficult to feed back thermal errors in time, etc. question
- Summary
- Abstract
- Description
- Claims
- Application Information
AI Technical Summary
Problems solved by technology
Method used
Image
Examples
Embodiment Construction
[0034] In order to make the technical problems, technical solutions and advantages to be solved by the present invention clearer, the present invention will be further described below in conjunction with the accompanying drawings and specific embodiments.
[0035] The invention establishes a relationship model between the bearing pretightening force of the electric spindle and the thermal error of the nose end of the electric spindle, which is mainly used for the prediction modeling of the different bearing pretightening force and the axial thermal error of the electric spindle under different working conditions, and improves the Modeling speed and generalization.
[0036] This embodiment is based on figure 1 The electric spindle bearing variable preload structure shown in the figure includes a pressurized gas channel (1), a bearing slide (2), a bearing (3) and a main shaft (4), and (5) in the figure is pressurized gas. A gas channel is provided in the pressure gas flow chann...
PUM
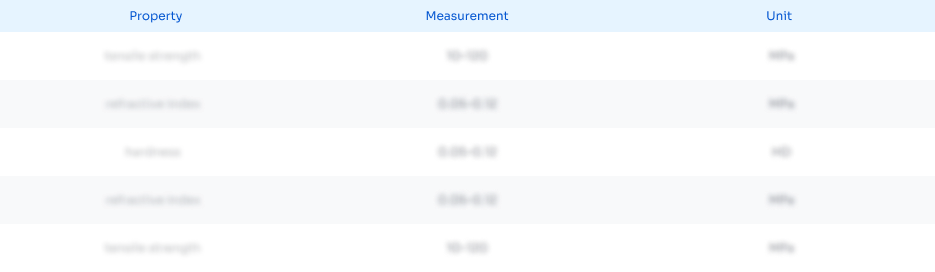
Abstract
Description
Claims
Application Information
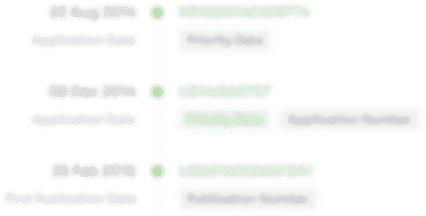
- R&D Engineer
- R&D Manager
- IP Professional
- Industry Leading Data Capabilities
- Powerful AI technology
- Patent DNA Extraction
Browse by: Latest US Patents, China's latest patents, Technical Efficacy Thesaurus, Application Domain, Technology Topic.
© 2024 PatSnap. All rights reserved.Legal|Privacy policy|Modern Slavery Act Transparency Statement|Sitemap