Rayleigh wave seismic data noise removal method, storage medium and electronic equipment
A technology of seismic data and data, applied in the field of engineering geophysical exploration, can solve the problems of artificial adjustment of parameters and application limitations, and achieve the effect of improving denoising efficiency, reducing labor cost and good denoising effect.
- Summary
- Abstract
- Description
- Claims
- Application Information
AI Technical Summary
Problems solved by technology
Method used
Image
Examples
no. 1 example
[0089] figure 1 is a schematic flow chart of the method for removing noise from Rayleigh wave seismic data in this embodiment;
[0090] figure 2 is a schematic diagram of the deep convolution generation confrontation network structure of this embodiment;
[0091] image 3 is the Rayleigh wave seismic data containing high random noise of the present embodiment;
[0092] Figure 4 It is the Rayleigh wave seismic data after DCGAN denoising of the present embodiment;
[0093] Figure 5 is the Rayleigh wave dispersion energy diagram containing high random noise of the present embodiment;
[0094] Figure 6 is the Rayleigh wave dispersion energy map after DCGAN denoising in this embodiment.
[0095] This embodiment provides a method for removing noise from Rayleigh wave seismic data, comprising the following steps:
[0096] Constructing a deep convolutional generation confrontation network, which includes a generator and a discriminator;
[0097] Preprocessing the Rayleig...
no. 2 example
[0172] figure 1 is a schematic flow chart of the method for removing noise from Rayleigh wave seismic data in this embodiment;
[0173] figure 2 is a schematic diagram of the deep convolution generation confrontation network structure of this embodiment;
[0174] image 3 is the Rayleigh wave seismic data containing high random noise of the present embodiment;
[0175] Figure 4 It is the Rayleigh wave seismic data after DCGAN denoising of the present embodiment;
[0176] Figure 5 is the Rayleigh wave dispersion energy diagram containing high random noise of the present embodiment;
[0177] Figure 6 is the Rayleigh wave dispersion energy map after DCGAN denoising in this embodiment.
[0178] This embodiment provides a method for removing noise from Rayleigh wave seismic data, comprising the following steps:
[0179] Constructing a deep convolutional generation confrontation network, which includes a generator and a discriminator;
[0180] Preprocessing the Rayleig...
no. 3 example
[0256] figure 1 is a schematic flow chart of the method for removing noise from Rayleigh wave seismic data in this embodiment;
[0257] figure 2 is a schematic diagram of the deep convolution generation confrontation network structure of this embodiment;
[0258] image 3 is the Rayleigh wave seismic data containing high random noise of the present embodiment;
[0259] Figure 4 It is the Rayleigh wave seismic data after DCGAN denoising of the present embodiment;
[0260] Figure 5 is the Rayleigh wave dispersion energy diagram containing high random noise of the present embodiment;
[0261] Figure 6 is the Rayleigh wave dispersion energy map after DCGAN denoising in this embodiment.
[0262] This embodiment provides a method for removing noise from Rayleigh wave seismic data, comprising the following steps:
[0263] Constructing a deep convolutional generation confrontation network, which includes a generator and a discriminator;
[0264] Preprocessing the Rayleig...
PUM
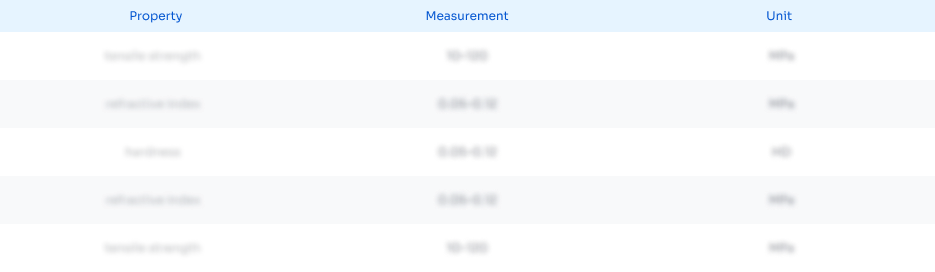
Abstract
Description
Claims
Application Information
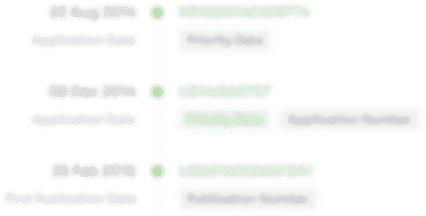
- R&D Engineer
- R&D Manager
- IP Professional
- Industry Leading Data Capabilities
- Powerful AI technology
- Patent DNA Extraction
Browse by: Latest US Patents, China's latest patents, Technical Efficacy Thesaurus, Application Domain, Technology Topic, Popular Technical Reports.
© 2024 PatSnap. All rights reserved.Legal|Privacy policy|Modern Slavery Act Transparency Statement|Sitemap|About US| Contact US: help@patsnap.com