Deep network model for radar signal sorting
A radar signal sorting and deep network technology, applied in biological neural network models, character and pattern recognition, instruments, etc., can solve the problems of poor sorting accuracy and low efficiency, and achieve strong robustness, high efficiency, and high efficiency The effect of sorting work
- Summary
- Abstract
- Description
- Claims
- Application Information
AI Technical Summary
Problems solved by technology
Method used
Image
Examples
Embodiment Construction
[0014] The technical solutions of the present invention will be described in detail below in conjunction with the accompanying drawings and preferred embodiments.
[0015] Such as figure 1 As shown, this embodiment provides a deep network model for radar signal sorting, which includes a radar signal sorting network based on a convolutional neural network and a long-short-term memory network obtained after training a pre-trained model using a training set RadarNet, where the pre-training model has the same network structure as the radar signal sorting network RadarNet. The radar signal sorting network RadarNet includes a local feature learning sub-network using a convolutional neural network (CNN) as the main structure, a cross-domain feature learning sub-network using a long-short-term memory network (LSTM) as the main structure, a feature fusion module, and a classifier. .
[0016] The input of the radar signal sorting network RadarNet is the feature vector. The radar signa...
PUM
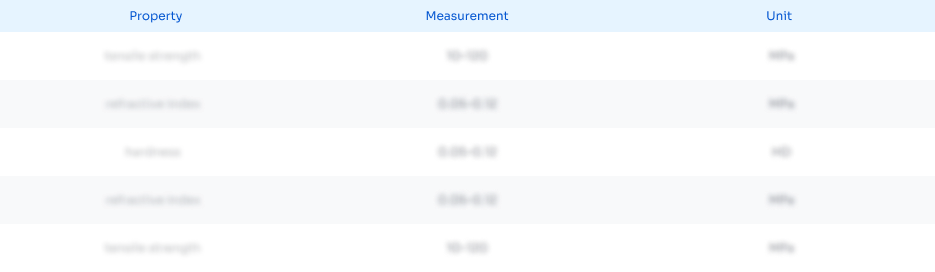
Abstract
Description
Claims
Application Information
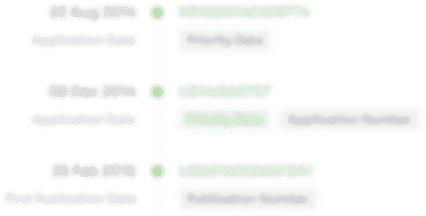
- R&D Engineer
- R&D Manager
- IP Professional
- Industry Leading Data Capabilities
- Powerful AI technology
- Patent DNA Extraction
Browse by: Latest US Patents, China's latest patents, Technical Efficacy Thesaurus, Application Domain, Technology Topic, Popular Technical Reports.
© 2024 PatSnap. All rights reserved.Legal|Privacy policy|Modern Slavery Act Transparency Statement|Sitemap|About US| Contact US: help@patsnap.com