Hierarchical nonlinear subspace dictionary learning method for remote sensing image scene classification
A dictionary learning and scene classification technology, applied in the field of remote sensing image processing, can solve the problems of time-consuming, classification performance disaster, dictionary learning memory usage and increased computational complexity, and achieve good classification performance
- Summary
- Abstract
- Description
- Claims
- Application Information
AI Technical Summary
Problems solved by technology
Method used
Image
Examples
Embodiment Construction
[0087] The technical solution of the present invention will be further described in detail below in conjunction with the accompanying drawings.
[0088] The present invention proposes a hierarchical nonlinear subspace dictionary learning (Hierarchical nonlinear subspace dictionary learning, HNSDL) method. A large number of literatures show that spatial information can improve the accuracy of remote sensing image classification. Inspired by multi-layer dictionary learning, HNSDL uses a hierarchical nonlinear method to project data samples into a series of subspaces and achieve data dimensionality reduction. In order to improve the discriminative ability of the model, a local structure constraint term of sparse coding is introduced in the learning process. Experiments on multiple real remote sensing image datasets show that the proposed method is effective for remote sensing image scene classification. Specifically, the main contributions of the present invention can be summar...
PUM
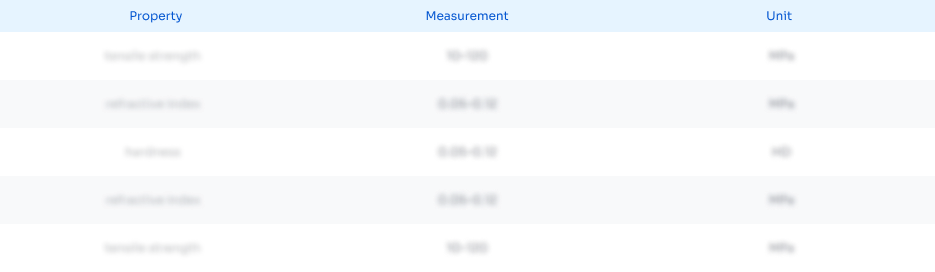
Abstract
Description
Claims
Application Information
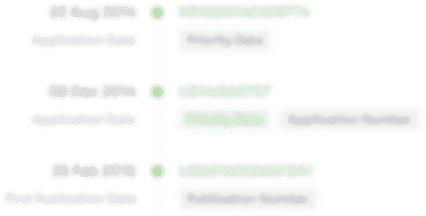
- R&D Engineer
- R&D Manager
- IP Professional
- Industry Leading Data Capabilities
- Powerful AI technology
- Patent DNA Extraction
Browse by: Latest US Patents, China's latest patents, Technical Efficacy Thesaurus, Application Domain, Technology Topic, Popular Technical Reports.
© 2024 PatSnap. All rights reserved.Legal|Privacy policy|Modern Slavery Act Transparency Statement|Sitemap|About US| Contact US: help@patsnap.com