Wind power variable pitch system fault diagnosis method based on CEEMDAN-BNs
A technology of system faults and diagnostic methods, which is applied in wind turbines, monitoring of wind turbines, complex mathematical operations, etc., can solve the complex establishment of Bayesian networks, the limitations of Bayesian network applications, and the acquisition of conditional probability distributions of Bayesian networks Difficulty and other problems, to achieve the effect of improving endpoint effects, improving modal aliasing, and reducing training time
- Summary
- Abstract
- Description
- Claims
- Application Information
AI Technical Summary
Problems solved by technology
Method used
Image
Examples
Embodiment
[0069] Such as figure 1 Shown, a kind of wind power variable pitch system fault diagnosis method based on CEEMDAN-BNs of the present invention comprises the following steps:
[0070] S1. Add white noise to the sample time series signal of the fault state, perform CEEMDAN modal decomposition, and obtain IMF and margin signals of multiple modal component signals.
[0071] The simulation model of the wind turbine is monitored, the vibration signal in the fault state is collected, the collected signal is divided and preprocessed, and the sample time series signal of the fault state is obtained.
[0072] Adaptive Complete Empirical Mode Decomposition of Noise (CEEMDAN), as an adaptive and completely non-recursive time-frequency analysis method, can decompose multi-component signals into multiple single-component signals at one time, and use iterative search to adaptively match when solving The center frequency and effective bandwidth of each single-component signal effectively sup...
PUM
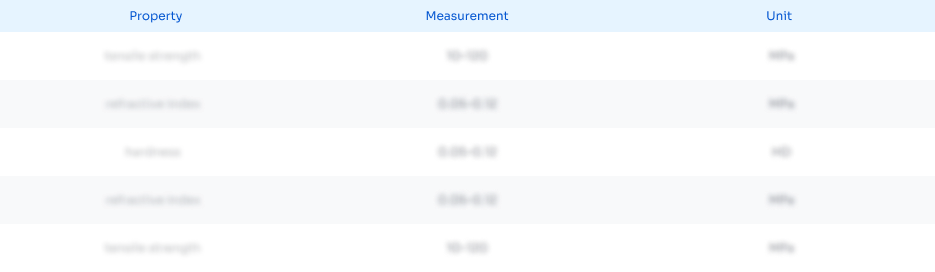
Abstract
Description
Claims
Application Information
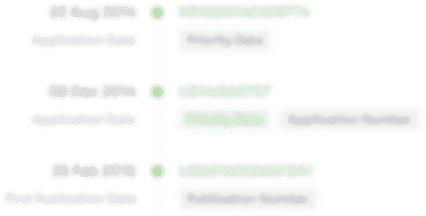
- R&D Engineer
- R&D Manager
- IP Professional
- Industry Leading Data Capabilities
- Powerful AI technology
- Patent DNA Extraction
Browse by: Latest US Patents, China's latest patents, Technical Efficacy Thesaurus, Application Domain, Technology Topic, Popular Technical Reports.
© 2024 PatSnap. All rights reserved.Legal|Privacy policy|Modern Slavery Act Transparency Statement|Sitemap|About US| Contact US: help@patsnap.com