Spacecraft attitude control method based on deep neural network approximation MPC
A deep neural network and attitude control technology, applied in attitude control, vehicle position/route/height control, control/adjustment system, etc., can solve problems such as difficult to determine the scale of neural network, unsuitable for spacecraft, difficult training, etc., to achieve The effect of improving online control efficiency, avoiding constraint violations, and improving the time required for training
- Summary
- Abstract
- Description
- Claims
- Application Information
AI Technical Summary
Problems solved by technology
Method used
Image
Examples
Embodiment Construction
[0056] The present invention will be further described below in conjunction with the accompanying drawings and specific preferred embodiments, but the protection scope of the present invention is not limited thereby.
[0057] Such as figure 1 As shown, the steps of the spacecraft attitude control method based on deep neural network approaching MPC in this embodiment include:
[0058] Step S01. Training data set generation: Configure the MPC controller based on the model predictive control method. The MPC controller inputs the attitude parameters of the spacecraft and outputs the desired control torque for the spacecraft. The attitude parameters include attitude errors or are obtained by converting attitude errors. Parameters, the attitude error is the error value between the current attitude parameter value and the expected attitude parameter value of the spacecraft, the control inputs multiple input attitude parameters into the MPC controller, and obtains corresponding multi...
PUM
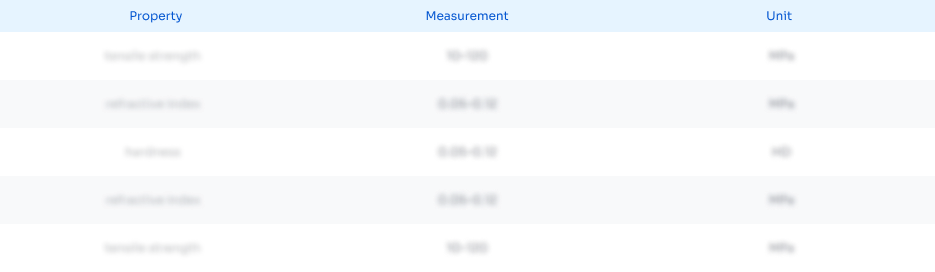
Abstract
Description
Claims
Application Information
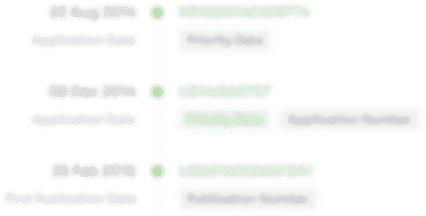
- R&D Engineer
- R&D Manager
- IP Professional
- Industry Leading Data Capabilities
- Powerful AI technology
- Patent DNA Extraction
Browse by: Latest US Patents, China's latest patents, Technical Efficacy Thesaurus, Application Domain, Technology Topic, Popular Technical Reports.
© 2024 PatSnap. All rights reserved.Legal|Privacy policy|Modern Slavery Act Transparency Statement|Sitemap|About US| Contact US: help@patsnap.com