Multi-target-domain equipment fault diagnosis method based on AdaDCLF
A technology for equipment failure and diagnosis methods, applied in neural learning methods, instruments, biological neural network models, etc., can solve problems such as the performance degradation of training models, and achieve the effect of enhancing generalization ability, improving diagnosis accuracy, and realizing intelligent diagnosis.
- Summary
- Abstract
- Description
- Claims
- Application Information
AI Technical Summary
Problems solved by technology
Method used
Image
Examples
specific Embodiment
[0080] The original data set of the experiment has collected data under four different working conditions, with a total of 6000 samples. According to different working conditions, the data is divided into source domain and three target domain data. The source domain data has 3000 samples, and each target domain data has 1000 samples. The source domain data is labeled, and the target domain data is unlabeled. The source domain data is labeled 0, and the three target domain data are labeled 1 to 3. The source domain data is randomly divided into the original training set and the test set at a ratio of 4:1. The source domain data is used for training, and the AdaDCLF method is used to generalize the model on multiple target domains through the source domain and target domain data. Finally, the target domain data is input into the final model to obtain the final fault diagnosis result.
[0081] The experimental environment of the present invention is: CPU is CoreTMi7-6700K@4.00G...
PUM
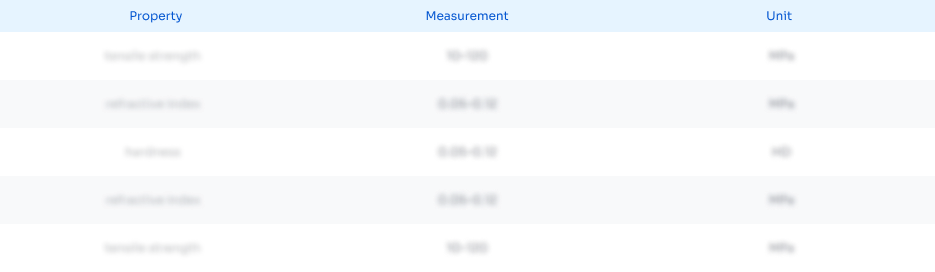
Abstract
Description
Claims
Application Information
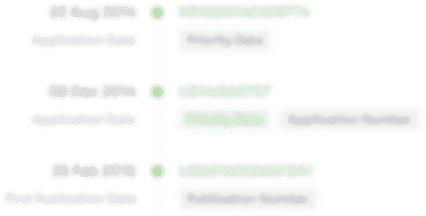
- R&D
- Intellectual Property
- Life Sciences
- Materials
- Tech Scout
- Unparalleled Data Quality
- Higher Quality Content
- 60% Fewer Hallucinations
Browse by: Latest US Patents, China's latest patents, Technical Efficacy Thesaurus, Application Domain, Technology Topic, Popular Technical Reports.
© 2025 PatSnap. All rights reserved.Legal|Privacy policy|Modern Slavery Act Transparency Statement|Sitemap|About US| Contact US: help@patsnap.com