Image sentiment analysis method based on sentiment attribute mining
A sentiment analysis and emotion technology, applied in semantic analysis, neural learning methods, instruments, etc., can solve the problems of difficult to deal with the complexity of image emotion semantic mapping relationship, lack of middle-level semantic representation, large amount of data set engineering, etc. The effect of strong discriminative ability, large semantic coverage, and large semantic coverage
- Summary
- Abstract
- Description
- Claims
- Application Information
AI Technical Summary
Problems solved by technology
Method used
Image
Examples
Embodiment 1
[0062] An image sentiment analysis method based on emotional attribute mining, using user metadata information mining to construct a concept selection model to realize the selection of emotional attributes, and further propose a fusion of neural network and matrix decomposition model to realize image label prediction based on user annotation, operation steps as follows:
[0063] Step 1: According to the characteristics of user tags and emotional attributes, construct a concept selection model that integrates four emotional attribute characteristics, and train the model to complete the selection of emotional attribute subsets;
[0064] Step 2: Use the convolutional neural network to extract visual features, and obtain the visual factor matrix by mapping the transformation matrix to the latent shared space, and optimize it through visual content consistency;
[0065] Step 3: Construct the adjacency graph guided by the external knowledge base, use the graph convolutional network ...
Embodiment 2
[0070] This embodiment is basically the same as the above-mentioned embodiment, and the special features are:
[0071] In the present embodiment, in the step 1, the concept selection model that combines four kinds of emotional attribute characteristics is constructed and the method for obtaining the emotional attribute subset is defined as a group that has obvious effects on emotional transmission in the semantic part of the image cognitive layer. Contributed visual semantic concepts, the four emotional attribute characteristics are semantic modelability, emotional discriminability, semantic coverage and limitedness. The specific definitions and calculation methods are:
[0072] 1) Semantic modelability, using the calculation method of information entropy, using the intra-cluster difference as the probability weight of each cluster, adding inter-cluster difference to amplify the image cluster information entropy of the concept, and calculating the semantic modelability, The sp...
Embodiment 3
[0112] This embodiment is basically the same as the above-mentioned embodiment, and the special features are:
[0113] figure 1 A flow chart of an image sentiment analysis method based on sentiment attribute mining disclosed by the present invention is shown, as figure 1 As shown, the method includes the following steps:
[0114] Step 1: According to the characteristics of user tags and emotional attributes, construct a concept selection model that integrates four emotional attribute characteristics, and train the model to complete the selection of emotional attribute subsets, specifically including the following steps:
[0115] 1) Calculate the semantic modelability through the intra-cluster visual difference and inter-cluster visual difference of the associated image clusters of each concept, the specific steps are as follows:
[0116] 1-1) For each concept c in the concept set C, through the associated label feature set of the image Calculate c and i image Semantic sim...
PUM
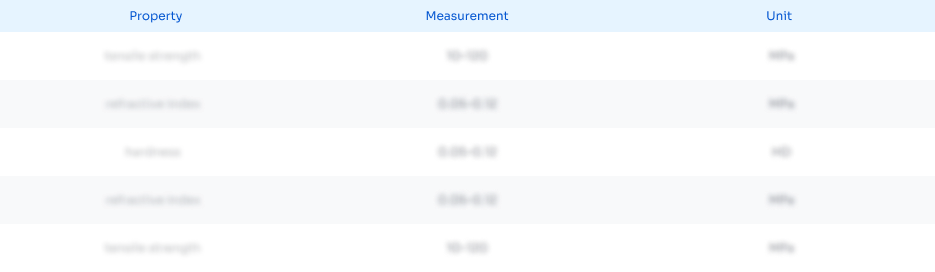
Abstract
Description
Claims
Application Information
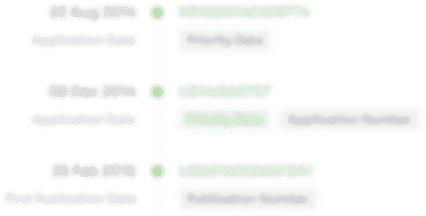
- R&D Engineer
- R&D Manager
- IP Professional
- Industry Leading Data Capabilities
- Powerful AI technology
- Patent DNA Extraction
Browse by: Latest US Patents, China's latest patents, Technical Efficacy Thesaurus, Application Domain, Technology Topic, Popular Technical Reports.
© 2024 PatSnap. All rights reserved.Legal|Privacy policy|Modern Slavery Act Transparency Statement|Sitemap|About US| Contact US: help@patsnap.com