Motor imagery electroencephalogram signal classification method based on channel attention and multi-scale time domain convolution
An EEG signal and motor imagery technology, applied in character and pattern recognition, medical science, instruments, etc., can solve the problems of low signal-to-noise ratio of EEG signals and difficulty in feature extraction, so as to improve training speed, maintain network performance, The effect of improving accuracy
- Summary
- Abstract
- Description
- Claims
- Application Information
AI Technical Summary
Problems solved by technology
Method used
Image
Examples
Embodiment Construction
[0020] Aiming at the problem of difficult feature extraction and classification caused by low signal-to-noise ratio of EEG signals, the present invention proposes a motor imagery EEG signal classification method based on channel attention and multi-scale temporal convolution. The parallel multi-scale temporal convolutional layer is used to replace the ordinary convolutional layer in the EEGNet model for better feature extraction, thereby improving classification accuracy. At the same time, the channel attention module ECA is added to make the network training pay more attention to the channel information with high correlation with the input data, further improve the robustness of the model, and provide an efficient and better performance deep learning method for motor imagery EEG signal classification .
[0021] figure 1 For the general flowchart of the inventive method, can be decomposed into following several steps:
[0022] Step 1. Data preprocessing, and divide the data ...
PUM
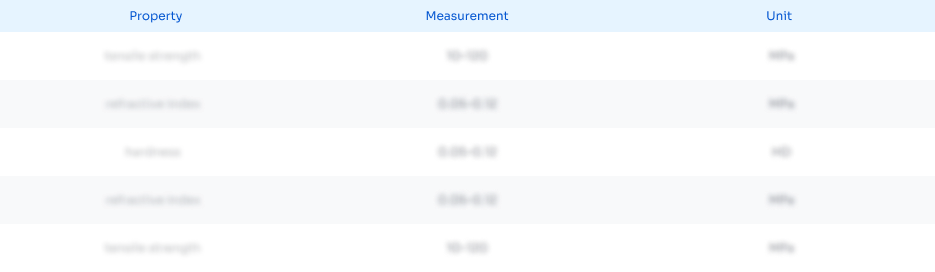
Abstract
Description
Claims
Application Information
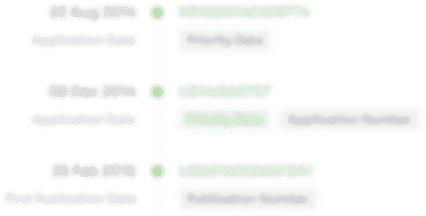
- R&D
- Intellectual Property
- Life Sciences
- Materials
- Tech Scout
- Unparalleled Data Quality
- Higher Quality Content
- 60% Fewer Hallucinations
Browse by: Latest US Patents, China's latest patents, Technical Efficacy Thesaurus, Application Domain, Technology Topic, Popular Technical Reports.
© 2025 PatSnap. All rights reserved.Legal|Privacy policy|Modern Slavery Act Transparency Statement|Sitemap|About US| Contact US: help@patsnap.com