MRI image and CT image conversion method and terminal based on deep learning
A CT image and deep learning technology, applied in the field of medical image processing, can solve problems such as inaccurate feature extraction, long inspection time, and poor contrast in soft tissue imaging, so as to avoid redundant model design, improve image conversion efficiency, and avoid noise influence Effect
- Summary
- Abstract
- Description
- Claims
- Application Information
AI Technical Summary
Problems solved by technology
Method used
Image
Examples
Embodiment 1
[0085] Please refer to figure 1 , a method for converting MRI images and CT images based on deep learning in this embodiment, including:
[0086] S1. Obtain a training MRI image and a training CT image, and perform N4 offset correction and histogram matching on the training MRI image and the training CT image to obtain a preprocessed training MRI image and a preprocessed training CT image;
[0087] In S1, N4 offset correction and histogram matching are performed on the training MRI image and the training CT image, and the preprocessed training MRI image and the preprocessed training CT image include:
[0088] S11. Obtain a training MRI grayscale image and a training CT grayscale image according to the training MRI image and the training CT image;
[0089] Specifically, set corresponding file paths for the training MRI image source_A and the training CT image source_B respectively, cut the training MRI image source_A and the training CT image source_B into the same size, name th...
Embodiment 2
[0152] Please refer to figure 1 , 3 -6. On the basis of Embodiment 1, this embodiment further defines how to train to obtain the first final full convolutional neural network model and the second final full convolutional neural network model, specifically:
[0153] Step S2 is specifically:
[0154] S21. Input the preprocessed training MRI image into the encoder network of the initial fully convolutional neural network model to obtain a first low-resolution feature map and a first high-resolution feature map;
[0155] Wherein, the encoder network includes a first convolutional layer, a first activation layer, a second convolutional layer, a second activation layer, a maximum pooling layer, a third activation layer and a downsampling layer arranged in sequence;
[0156] Specifically, input the preprocessed training MRI image into the encoder network of the initial full convolutional neural network model, and then pass through the first convolutional layer, the first activation...
Embodiment 3
[0241] The difference between this embodiment and Embodiment 1 or Embodiment 2 is that some specific parameters for training the initial fully convolutional neural network model are further limited:
[0242] Specify the GPU, choose single card or multiple cards, choose according to the image data size, that is, os.environ['CUDA_VISIBLE_DEVICES']=GPU_id;
[0243] The image batch size Batch_size is set to 8;
[0244] The learning rate learning_rate is set to 0.0001 (1e-4);
[0245] The decay factor weight_decay is set to 1e-4;
[0246] The preset training round epoch is set to 600;
[0247] Save the model save_model is set to every 50epoch;
[0248] The error output of training, verification and testing is MAE (Mean Absolute Error, average absolute error), ME (Mean Error, average error), MSE (Mean Squared Error, mean square error) and PCC (PearsonCorrelation Coefficient, Pearson correlation coefficient) , through multiple measures of MAE, ME, MSE and PCC, multiple measuremen...
PUM
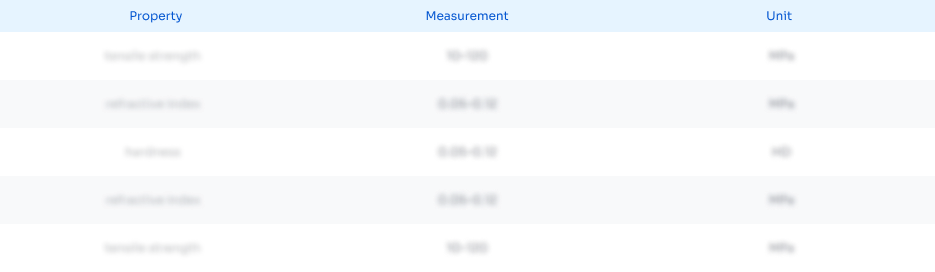
Abstract
Description
Claims
Application Information
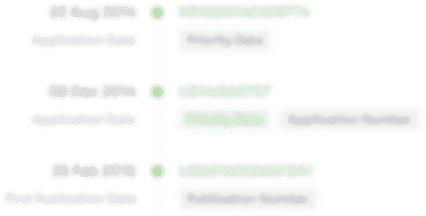
- R&D Engineer
- R&D Manager
- IP Professional
- Industry Leading Data Capabilities
- Powerful AI technology
- Patent DNA Extraction
Browse by: Latest US Patents, China's latest patents, Technical Efficacy Thesaurus, Application Domain, Technology Topic, Popular Technical Reports.
© 2024 PatSnap. All rights reserved.Legal|Privacy policy|Modern Slavery Act Transparency Statement|Sitemap|About US| Contact US: help@patsnap.com