Robot reality migration system and method based on reinforcement learning and residual error modeling
A technology of reinforcement learning and transfer systems, applied in neural learning methods, biological models, instruments, etc., can solve the problems of high-performance expert data collection difficulties, poor execution, low sampling efficiency, etc., to speed up convergence and generalization , wide application prospects, the effect of strong generalization ability
- Summary
- Abstract
- Description
- Claims
- Application Information
AI Technical Summary
Problems solved by technology
Method used
Image
Examples
Embodiment Construction
[0046] Below in conjunction with specific embodiment, further illustrate the present invention, should be understood that these embodiments are only used to illustrate the present invention and are not intended to limit the scope of the present invention, after having read the present invention, those skilled in the art will understand various equivalent forms of the present invention All modifications fall within the scope defined by the appended claims of the present application.
[0047] Aiming at the problem that the behavior policy obtained by using reinforcement learning to train the robot in the simulator does not perform well in the actual scene, which leads to the problem that the robot based on reinforcement learning training cannot be applied, a robot based on reinforcement learning and residual modeling is proposed. Reality transfer system and method. The robot reality transfer system based on reinforcement learning and residual modeling includes environment simula...
PUM
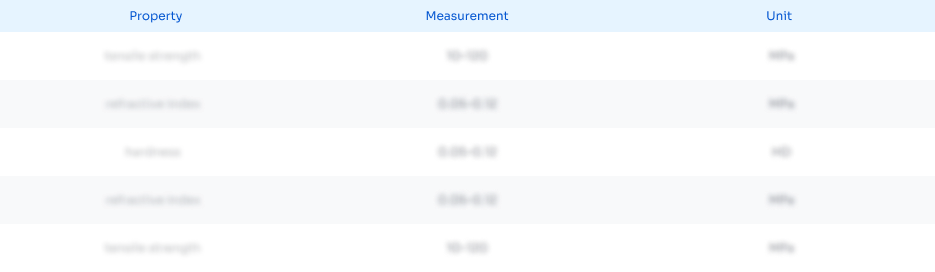
Abstract
Description
Claims
Application Information
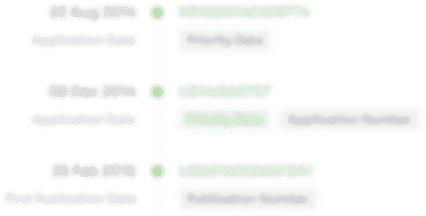
- R&D Engineer
- R&D Manager
- IP Professional
- Industry Leading Data Capabilities
- Powerful AI technology
- Patent DNA Extraction
Browse by: Latest US Patents, China's latest patents, Technical Efficacy Thesaurus, Application Domain, Technology Topic.
© 2024 PatSnap. All rights reserved.Legal|Privacy policy|Modern Slavery Act Transparency Statement|Sitemap