Underwater group target detection method fusing YOLOv4 and deformable convolution
A target detection and group technology, applied in neural learning methods, instruments, biological neural network models, etc., can solve the problems of difficulty in ensuring the accuracy and recall rate of underwater groups, easy to miss detection, complex network structure, etc., to alleviate the problem. The effect of dense target occlusion, flexible threshold selection, and optimized loss function
- Summary
- Abstract
- Description
- Claims
- Application Information
AI Technical Summary
Problems solved by technology
Method used
Image
Examples
Embodiment Construction
[0026] An underwater group target detection method that combines YOLOv4 and deformable convolution of the present invention is the same as the prior art, and is to send the target image of the underwater aquaculture group to be detected into the network model for detection and use the NMS algorithm to screen, To obtain the location and classification information of the underwater culture population, the difference from the prior art is that the network model is constructed according to the following steps:
[0027] Step 1. Collect the video data of the underwater aquaculture group target in the real aquaculture environment, and perform preprocessing such as frame extraction and screening on the video data to obtain the target picture of the underwater aquaculture group; specifically, obtain the real aquatic product from Dalian Tianzheng Industrial Co., Ltd. For the video data of farmed products in the breeding environment, through the video frame extraction program, a picture i...
PUM
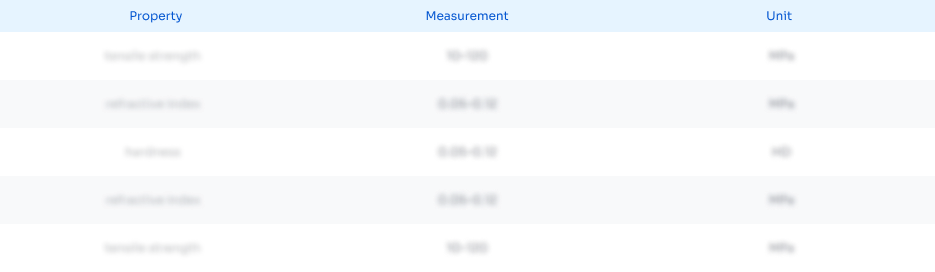
Abstract
Description
Claims
Application Information
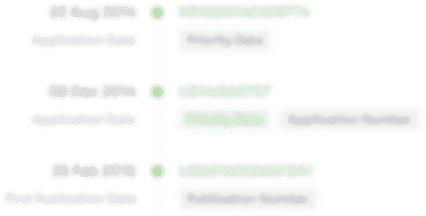
- R&D Engineer
- R&D Manager
- IP Professional
- Industry Leading Data Capabilities
- Powerful AI technology
- Patent DNA Extraction
Browse by: Latest US Patents, China's latest patents, Technical Efficacy Thesaurus, Application Domain, Technology Topic.
© 2024 PatSnap. All rights reserved.Legal|Privacy policy|Modern Slavery Act Transparency Statement|Sitemap