Assessment of antigen repair and target repair progression quantitation using vibration spectroscopy
A technique of vibrational spectroscopy, spectral data, applied in the field of quantitative assessment of antigen retrieval and target retrieval progress using vibrational spectroscopy, which can solve problems such as no general methods or reagents have been identified.
- Summary
- Abstract
- Description
- Claims
- Application Information
AI Technical Summary
Problems solved by technology
Method used
Image
Examples
example 1
[0158] Example 1 – Estimate unmasked state using trained unmasked state estimation engine
[0159] the summary of instruction
[0160] This project uses mid-infrared (mid-IR) spectroscopy to study the vibrational states of molecules in histological tissue sections. The testable hypothesis is that the vibrational state of tissue, as judged by the mid-infrared signal, will exhibit conformational changes in tissue composition that occur during antigen retrieval (AR).
[0161] A metrology has been developed that correlates changes in the mid-infrared spectrum with the antigen retrieval status of tissues. This metrology was used to examine the difference between low temperature and high temperature repairs. Mid-infrared results were confirmed by staining results showing low and high temperature antigen retrieval resulting in different staining intensities, suggesting that these treatments do not leave the tissue in an equivalent state.
[0162] In this work, changes in the m...
example 2
[0201] Example 2 – Training an Unmasked State Estimation Engine
[0202] In one embodiment, the repair status of the tissue is determined using a principal component analysis plus discriminant analysis (PCDA) algorithm. Mid-infrared spectra were collected from approximately one hundred locations throughout the tissue sample, so the average spectrum represents an average for the tissue. All spectra were atmospherically corrected to remove CO2 pollution, baseline corrected using concave rubber band correction with 10 iterations and 64 baseline points, and acquired spectra were amplitude normalized. Finally, all spectra from each tissue were averaged together. Next, use the PCDA model to classify the group to which a given spectrum belongs. The two main variables in this algorithm are the number of principal components used for classification purposes and the type of discriminant analysis (linear or quadratic). An example is shown in Figure 7A, where the first two principal ...
PUM
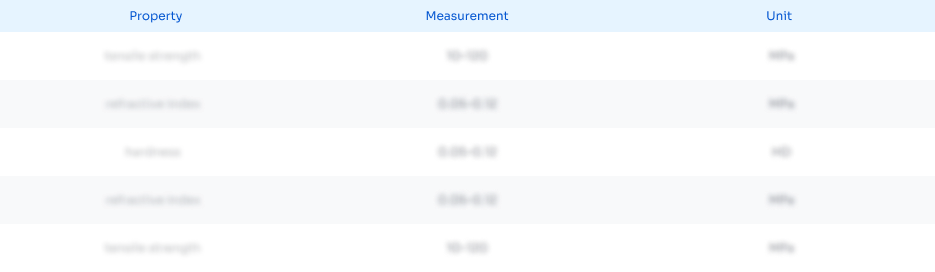
Abstract
Description
Claims
Application Information
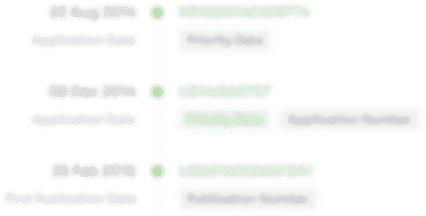
- R&D
- Intellectual Property
- Life Sciences
- Materials
- Tech Scout
- Unparalleled Data Quality
- Higher Quality Content
- 60% Fewer Hallucinations
Browse by: Latest US Patents, China's latest patents, Technical Efficacy Thesaurus, Application Domain, Technology Topic, Popular Technical Reports.
© 2025 PatSnap. All rights reserved.Legal|Privacy policy|Modern Slavery Act Transparency Statement|Sitemap|About US| Contact US: help@patsnap.com