New energy power system elastic optimization method based on deep reinforcement learning
A power system and reinforcement learning technology, applied in neural learning methods, constraint-based CAD, system integration technology, etc., can solve the problems that cannot meet the intelligence and flexibility requirements of new energy grids, and meet economic indicators and reliability Sexual indicators, reducing environmental pollution, and improving overall efficiency
- Summary
- Abstract
- Description
- Claims
- Application Information
AI Technical Summary
Problems solved by technology
Method used
Image
Examples
Embodiment Construction
[0054] The present invention will be described in detail below with reference to the accompanying drawings and examples.
[0055] Aiming at the problems existing in the traditional power system using hierarchical management and hierarchical scheduling for active power scheduling, the present invention proposes a new energy power system elastic optimization method based on deep reinforcement learning, and adopts an active power scheduling method based on deep reinforcement learning. The method greatly reduces the labor cost generated in the dispatching process, and also avoids the influence brought by the delay of manual communication.
[0056] In order to solve the above technical problems, the present invention adopts a new energy power system elasticity optimization method based on deep reinforcement learning, figure 1 A schematic structural diagram of the system is shown. The present invention adopts a deep reinforcement learning method. According to the theory of deep rein...
PUM
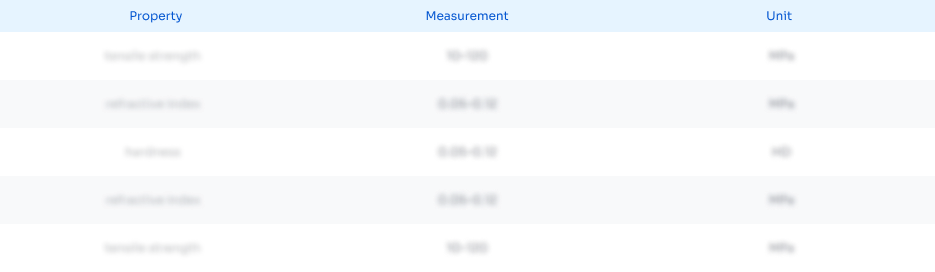
Abstract
Description
Claims
Application Information
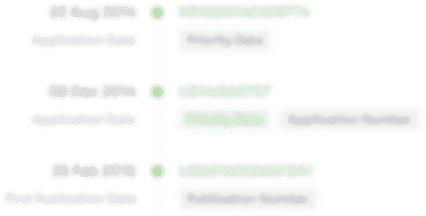
- R&D Engineer
- R&D Manager
- IP Professional
- Industry Leading Data Capabilities
- Powerful AI technology
- Patent DNA Extraction
Browse by: Latest US Patents, China's latest patents, Technical Efficacy Thesaurus, Application Domain, Technology Topic, Popular Technical Reports.
© 2024 PatSnap. All rights reserved.Legal|Privacy policy|Modern Slavery Act Transparency Statement|Sitemap|About US| Contact US: help@patsnap.com