Multi-step prediction method for clean coal quality in dense medium coal separation process based on LSTM (Long Short Term Memory)
A multi-step forecasting and dense medium technology, applied in forecasting, neural learning methods, biological neural network models, etc., can solve the problem of large fluctuations in measurement results, a large degree of dependence on experience in the setting of sorting density, and difficulty in reflecting the quality of clean coal and other issues, to achieve the effects of stable product quality, precise control and stability, and good prediction accuracy
- Summary
- Abstract
- Description
- Claims
- Application Information
AI Technical Summary
Problems solved by technology
Method used
Image
Examples
Embodiment Construction
[0035] In order to make the technical solutions of the present invention clearer and clearer to those skilled in the art, the following examples and accompanying drawings are combined. The present invention will be described in further detail, but the embodiments of the present invention are not limited thereto.
[0036] like Figure 1-2 As shown, this embodiment provides a multi-step prediction method for clean coal quality in the dense medium coal preparation process based on LSTM. Through time reconstruction of input and output data, combined with the LSTM algorithm, the clean coal quality in the production process of the coal preparation plant can be realized. Online multi-step forecasting. Specific steps are as follows:
[0037] Step 1: Collect online sensor data related to coal preparation production, such as belt scale, ash meter, suspension density, liquid level value, pressure gauge value, etc., to form a historical database stored on a daily basis.
[0038] Step 2...
PUM
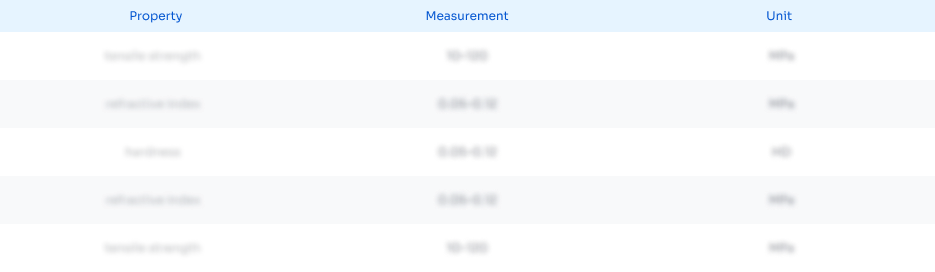
Abstract
Description
Claims
Application Information
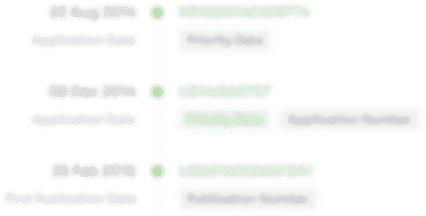
- R&D Engineer
- R&D Manager
- IP Professional
- Industry Leading Data Capabilities
- Powerful AI technology
- Patent DNA Extraction
Browse by: Latest US Patents, China's latest patents, Technical Efficacy Thesaurus, Application Domain, Technology Topic, Popular Technical Reports.
© 2024 PatSnap. All rights reserved.Legal|Privacy policy|Modern Slavery Act Transparency Statement|Sitemap|About US| Contact US: help@patsnap.com