A Multi-step Prediction Method of Mobile Communication Traffic
A mobile communication and multi-step forecasting technology, applied in wireless communication, special data processing applications, instruments, etc., can solve the problems of poor linear model adaptability and low prediction accuracy, reduce algorithm complexity, improve prediction accuracy, and solve blind sexual effect
- Summary
- Abstract
- Description
- Claims
- Application Information
AI Technical Summary
Problems solved by technology
Method used
Image
Examples
specific Embodiment approach 1
[0012] Specific embodiment one: a kind of multi-step prediction method of mobile communication traffic, this method is finished by the following steps:
[0013] Step 1: Carry out Fourier transform to mobile communication traffic data, use described Fourier spectrum analysis result as the prior knowledge of wavelet decomposition integration;
[0014] Step 2: According to the prior knowledge of the Fourier spectrum obtained in Step 1, the maximum overlap discrete wavelet transform algorithm is used to select the Haar wavelet base and the number of decomposition layers, and the wavelet decomposition is performed on the dialogue traffic sequence to obtain the components corresponding to the Fourier spectrum The trend item and each detail item;
[0015] Step 3: According to the prior knowledge obtained in Step 1, integrate the detailed items in Step 2 into periodic items;
[0016] Step 4: Use the product seasonal ARIMA model to predict the trend item obtained in step 2 and the per...
specific Embodiment approach 2
[0033] Specific implementation mode two: this implementation mode is an embodiment of specific implementation mode one:
specific Embodiment approach
[0035] 1. Carry out Fourier spectral analysis on the traffic data of a community in Harbin City, Heilongjiang Province, see figure 1 , the analysis process is: firstly the traffic sequence is sampled at equal intervals, the sampling period is 1 hour, and the unit of the traffic is Ireland (Erl); the result of the Fourier spectrum analysis shows the traffic time The sequence data has obvious periodic characteristics in the spectral components such as 6, 8, 12, 24, 84, and 168 hours, and the periodic components are arranged in order of amplitude from large to small, as follows: T = 24 hours, T = Spectrum components such as 12 hours, T=6 hours, T=8 hours, T=168 hours, T=84 hours, etc., see figure 2 . Using said spectral components as prior knowledge of wavelet decomposition;
[0036] Two, according to the above-mentioned prior knowledge of Fourier spectrum, adopt the maximum overlapping discrete wavelet transform algorithm described in step 2 in the specific embodiment one, ca...
PUM
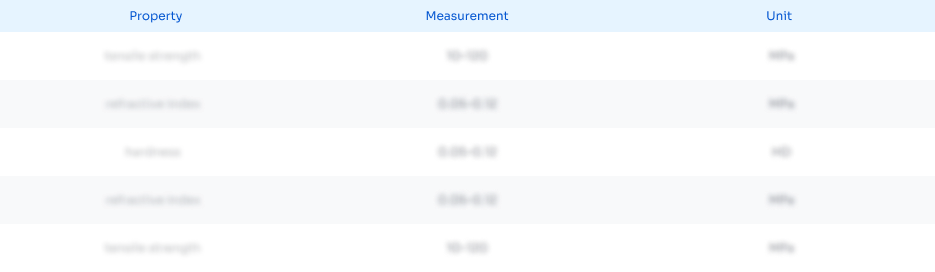
Abstract
Description
Claims
Application Information
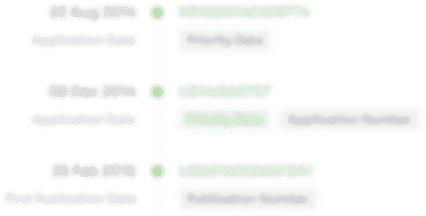
- R&D
- Intellectual Property
- Life Sciences
- Materials
- Tech Scout
- Unparalleled Data Quality
- Higher Quality Content
- 60% Fewer Hallucinations
Browse by: Latest US Patents, China's latest patents, Technical Efficacy Thesaurus, Application Domain, Technology Topic, Popular Technical Reports.
© 2025 PatSnap. All rights reserved.Legal|Privacy policy|Modern Slavery Act Transparency Statement|Sitemap|About US| Contact US: help@patsnap.com