Learning-based method for controlling unmanned aerial vehicle to complete trajectory tracking under wind disturbance
A trajectory tracking and unmanned aerial vehicle technology, applied in three-dimensional position/channel control, mechanical equipment, combustion engine, etc., can solve problems such as inability to achieve results, poor trajectory tracking accuracy, etc., to maintain fidelity, ensure real-time, The effect of improving efficiency
- Summary
- Abstract
- Description
- Claims
- Application Information
AI Technical Summary
Problems solved by technology
Method used
Image
Examples
Embodiment 1
[0071] Such as figure 1 As shown, a learning-based method for controlling UAV trajectory tracking under wind disturbance includes the following steps:
[0072] Step 1. Input the reference trajectory and UAV state, establish model predictive control based on the prior model, and optimize to obtain the corrected reference trajectory and reference control quantity.
[0073] Input the reference trajectory x, d, input the current state x of the drone; consider a nonlinear affine model
[0074] Transform the UAV system into this form; and linearize it to obtain a linear model, based on which model predictive control is established, that is, at each sampling time t k = t 0 +k×d t , t 0 is the current time, k is the number of prediction steps, and dt is the control interval, to solve a finite time-domain optimal control problem, as follows:
[0075]
[0076]
[0077]
[0078]
[0079]
[0080] u 1 (t)∈U
[0081] in To predict the state, the objective function...
Embodiment 2
[0129] This embodiment provides an electronic device, including: a memory, a processor, and a computer program stored on the memory and capable of running on the processor, and the processor executes the computer program to realize the learning-based learning described in Embodiment 1. The method of controlling UAV to complete trajectory tracking under wind disturbance.
Embodiment 3
[0131] This embodiment provides a computer-readable storage medium, on which a computer program is stored. When the computer program is executed by a processor, the learning-based control UAV described in Embodiment 1 is implemented to complete the trajectory under wind disturbance. method of tracking.
PUM
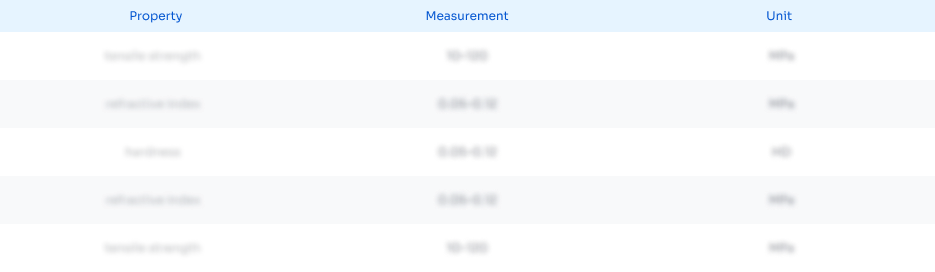
Abstract
Description
Claims
Application Information
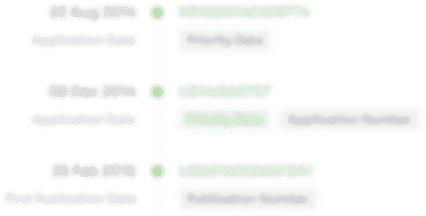
- R&D
- Intellectual Property
- Life Sciences
- Materials
- Tech Scout
- Unparalleled Data Quality
- Higher Quality Content
- 60% Fewer Hallucinations
Browse by: Latest US Patents, China's latest patents, Technical Efficacy Thesaurus, Application Domain, Technology Topic, Popular Technical Reports.
© 2025 PatSnap. All rights reserved.Legal|Privacy policy|Modern Slavery Act Transparency Statement|Sitemap|About US| Contact US: help@patsnap.com