Convolutional neural network model compression method, apparatus and device, and medium
A convolutional neural network and convolutional network technology, applied in biological neural network models, neural learning methods, neural architectures, etc., can solve problems such as DNN's inability to apply to storage and systems or equipment with limited computing power, and reduce computer costs. The effect of storage space, reducing its size, improving accuracy and performance
- Summary
- Abstract
- Description
- Claims
- Application Information
AI Technical Summary
Problems solved by technology
Method used
Image
Examples
Embodiment Construction
[0045] The embodiments of the present application provide a method for compressing a convolutional neural network model, which solves the technical problem in the prior art that complex DNNs cannot be applied to systems or devices with limited storage and computing capabilities.
[0046] The technical solution of the embodiment of the present application is to solve the above-mentioned technical problems, and the general idea is as follows:
[0047] A method for compressing a convolutional neural network model, the method comprising: setting a mask for each parameter group in each layer of the convolutional network model to be compressed, generating a model to be trained with a mask; wherein, the initial mask of each parameter group The values are all 1; N rounds of periodical training are performed on the model to be trained, where N is a positive integer, and a network sparse step is performed on the first model obtained from training. The network sparse step includes: dete...
PUM
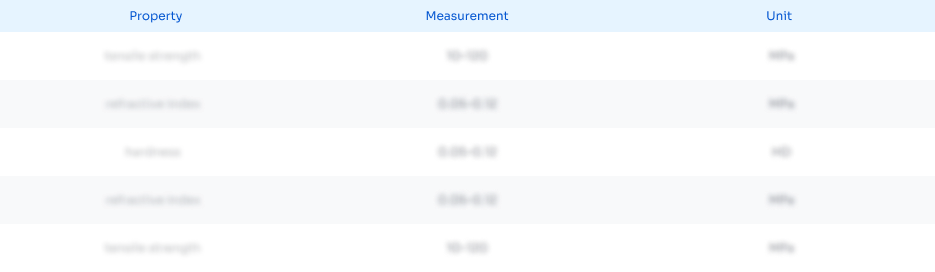
Abstract
Description
Claims
Application Information
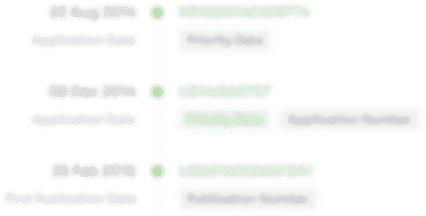
- R&D Engineer
- R&D Manager
- IP Professional
- Industry Leading Data Capabilities
- Powerful AI technology
- Patent DNA Extraction
Browse by: Latest US Patents, China's latest patents, Technical Efficacy Thesaurus, Application Domain, Technology Topic, Popular Technical Reports.
© 2024 PatSnap. All rights reserved.Legal|Privacy policy|Modern Slavery Act Transparency Statement|Sitemap|About US| Contact US: help@patsnap.com