Airship propeller reliability estimation method based on chaotic initialization SSA-BP neural network
A neural network and propeller technology, applied in the field of airship propeller reliability estimation, can solve problems such as poor strain detection, time-consuming finite element calculation, flight height and propeller speed cannot be accurately located at the design point, etc., to achieve rapid estimation and improvement Effects of Forecast Accuracy and Forecast Efficiency
- Summary
- Abstract
- Description
- Claims
- Application Information
AI Technical Summary
Problems solved by technology
Method used
Image
Examples
specific Embodiment
[0106] A method for estimating the reliability of an airship propeller based on a chaotic initialization SSA-BP neural network, comprising the following steps:
[0107] Determine the main factors affecting the blade strain under the design idle condition of the propeller;
[0108] Construct the training / test input data set of chaotic initialization SSA-BP neural network;
[0109] Solve the strain value of the maximum strain position of the propeller under the input data set as the working condition;
[0110] Establishment of chaos initialization SSA-BP neural network model;
[0111] Discretize the propeller design flight height and rotational speed under the mission profile with the 3σ principle to obtain a new input data set. Similarly, the propeller allowable strain value is discretized with the normal distribution according to the variation coefficient with the 3σ principle;
[0112] Solve the failure rate and mean time between failure (MTBF) of airship propeller.
[011...
PUM
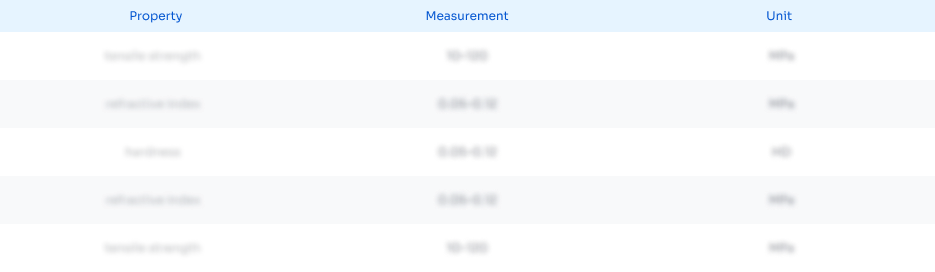
Abstract
Description
Claims
Application Information
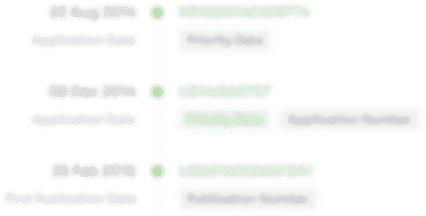
- R&D Engineer
- R&D Manager
- IP Professional
- Industry Leading Data Capabilities
- Powerful AI technology
- Patent DNA Extraction
Browse by: Latest US Patents, China's latest patents, Technical Efficacy Thesaurus, Application Domain, Technology Topic, Popular Technical Reports.
© 2024 PatSnap. All rights reserved.Legal|Privacy policy|Modern Slavery Act Transparency Statement|Sitemap|About US| Contact US: help@patsnap.com