Deep convolutional neural network quantization method, system and device, and storage medium
A neural network and deep convolution technology, applied in the field of deep learning, can solve the problems of not supporting channel-by-channel quantization, low quantization accuracy, and low efficiency in processing deep convolution neural networks, so as to improve the accuracy of quantitative reasoning and improve computing efficiency. Effect
- Summary
- Abstract
- Description
- Claims
- Application Information
AI Technical Summary
Problems solved by technology
Method used
Image
Examples
Embodiment Construction
[0021] In order to make the object, technical solution and advantages of the present invention clearer, the embodiments of the present invention will be further described in detail below in conjunction with specific embodiments and with reference to the accompanying drawings.
[0022] It should be noted that all expressions using "first" and "second" in the embodiments of the present invention are to distinguish two entities with the same name but different parameters or parameters that are not the same, see "first" and "second" It is only for the convenience of expression, and should not be construed as a limitation on the embodiments of the present invention, which will not be described one by one in the subsequent embodiments.
[0023] In the first aspect of the embodiments of the present invention, an embodiment of a method for quantizing a deep convolutional neural network is proposed. figure 1 What is shown is a schematic diagram of an embodiment of a method for quantizi...
PUM
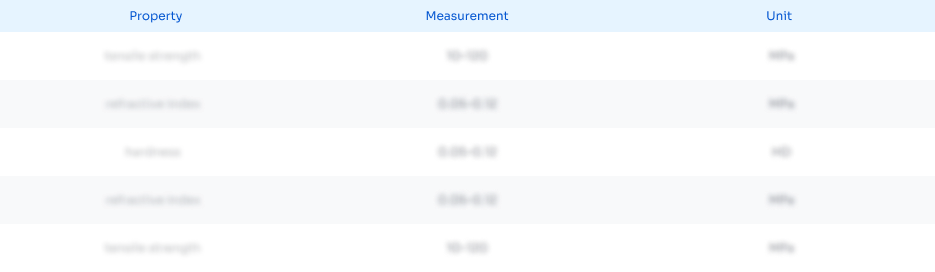
Abstract
Description
Claims
Application Information
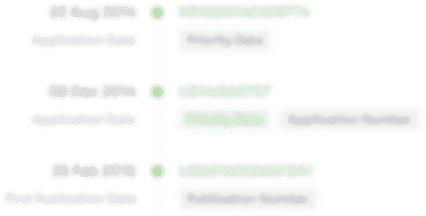
- R&D Engineer
- R&D Manager
- IP Professional
- Industry Leading Data Capabilities
- Powerful AI technology
- Patent DNA Extraction
Browse by: Latest US Patents, China's latest patents, Technical Efficacy Thesaurus, Application Domain, Technology Topic, Popular Technical Reports.
© 2024 PatSnap. All rights reserved.Legal|Privacy policy|Modern Slavery Act Transparency Statement|Sitemap|About US| Contact US: help@patsnap.com