Oil reservoir production prediction method based on graph wavelet neural network model
A technology of wavelet neural network and prediction method, applied in the direction of biological neural network model, neural learning method, prediction, etc., can solve the problems of interpretability and lack of information exchange between samples, large amount of calculation, and long time consumption, etc., to achieve good promotion Application value and effect of improving utilization efficiency
- Summary
- Abstract
- Description
- Claims
- Application Information
AI Technical Summary
Problems solved by technology
Method used
Image
Examples
Embodiment Construction
[0054] The present invention is described in further detail below in conjunction with the accompanying drawings and specific embodiments:
[0055] like figure 1 As shown, a method for predicting oil reservoir production based on a graph wavelet neural network model includes the following steps:
[0056] Step 1. Collect reservoir data, generate different reservoir production regimes, use numerical simulators to calculate the output of each production well under different production regimes, build a sample library, and divide the sample library into training sets and test sets in proportion. The specific process is:
[0057] Step 1.1. Collect reservoir data and generate 2000 groups of different reservoir production regimes.
[0058] Each set of production regimes includes injection well parameters and production well parameters. The injection well parameter is the water injection volume, and the production well parameter is the liquid production volume. The entire production...
PUM
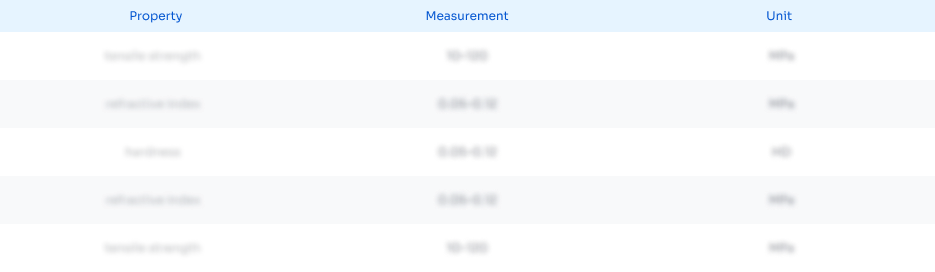
Abstract
Description
Claims
Application Information
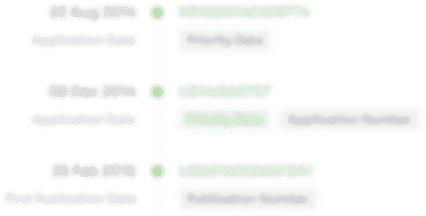
- R&D Engineer
- R&D Manager
- IP Professional
- Industry Leading Data Capabilities
- Powerful AI technology
- Patent DNA Extraction
Browse by: Latest US Patents, China's latest patents, Technical Efficacy Thesaurus, Application Domain, Technology Topic, Popular Technical Reports.
© 2024 PatSnap. All rights reserved.Legal|Privacy policy|Modern Slavery Act Transparency Statement|Sitemap|About US| Contact US: help@patsnap.com