Machine learning constraint-based density mutation interface inversion method and system
A machine learning, mutation interface technology, applied in the field of survey, can solve problems such as poor pertinence and affect the interpretability of the deep learning optimization process, and achieve the effect of enhancing accuracy and improving interpretability
- Summary
- Abstract
- Description
- Claims
- Application Information
AI Technical Summary
Problems solved by technology
Method used
Image
Examples
Embodiment 1
[0053] In the first embodiment, as figure 1 As shown, a method for inversion of density mutation interface based on machine learning constraints includes the following steps:
[0054] S1. According to the measured gravity data of the basin in the target area, the initial basin interface is determined by the Bouguer plate model. The calculation formula is as follows:
[0055]
[0056] in, is the initial depth of the basement of the basin model, For the measured gravity data, is the density difference between the surface and surrounding rock, is the gradient of density difference with depth; based on the initial basin interface, a variety of random transformation methods of probability distribution are used to generate multiple sets of basin disturbance interfaces.
[0057] S2. Perform a Hadamard product operation between the initial basin interface and the random basin disturbance interface data set to quickly generate a basin interface data set.
[0058] S3. Fill i...
Embodiment 2
[0076] In the second embodiment, a density mutation interface inversion system based on machine learning constraints includes: an interface generation module, a Hadamard product operation module, a function filling module, a forward modeling module, a migration imaging module, and a migration model optimization module Deep Learning Module and Gravity Multivariate Density Constrained Regular Inversion Module.
[0077] The interface generation module is used to construct an initial basin interface based on the measured gravity data, and randomly generate a perturbed basin interface dataset based on the initial basin interface.
[0078] The Hadamard product operation module is used to perform the Hadamard product operation based on the initial basin interface and disturbed basin interface data sets to obtain the basin interface data set.
[0079] The function filling module is used to fill in the density of the overlying strata based on the basin interface dataset to obtain a hig...
PUM
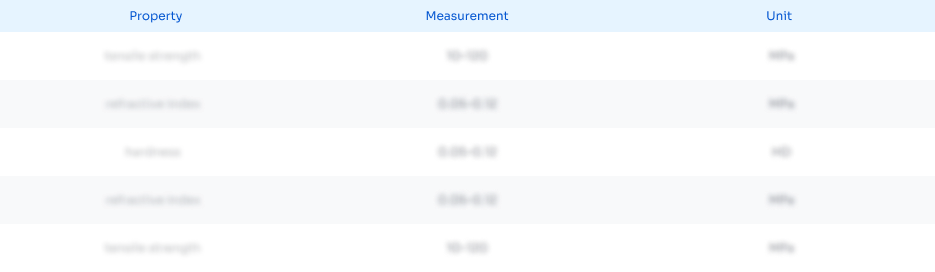
Abstract
Description
Claims
Application Information
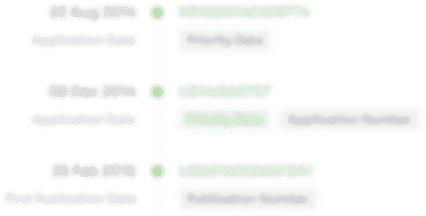
- R&D Engineer
- R&D Manager
- IP Professional
- Industry Leading Data Capabilities
- Powerful AI technology
- Patent DNA Extraction
Browse by: Latest US Patents, China's latest patents, Technical Efficacy Thesaurus, Application Domain, Technology Topic, Popular Technical Reports.
© 2024 PatSnap. All rights reserved.Legal|Privacy policy|Modern Slavery Act Transparency Statement|Sitemap|About US| Contact US: help@patsnap.com