Cloth defect detection method and system
A defect detection and cloth technology, applied in the field of computer vision, to achieve the effect of convenient subsequent segmentation, high computing efficiency, and enhanced reconstruction effect
- Summary
- Abstract
- Description
- Claims
- Application Information
AI Technical Summary
Problems solved by technology
Method used
Image
Examples
Embodiment 1
[0049] A cloth defect detection method, such as figure 1 shown, including the following steps:
[0050] S1. Input the cloth sample to be tested into the pre-trained lightweight generative adversarial network to obtain a reconstructed image of the cloth sample to be tested; the obtained reconstructed image is a defect-free image of the cloth sample to be tested.
[0051] Specifically, the training method for the above-mentioned lightweight generative adversarial network includes: duplicating the collected non-defective cloth sample set into two copies, and adding random noise to the samples in one of the non-defective cloth sample sets as a lightweight generative confrontation The input of the network, another non-defective cloth sample set does not do any processing, and is used as the output of the lightweight generative adversarial network, through the game between the light-weight generator and the discriminator, in order to fight against the light-weight generative adversa...
Embodiment approach
[0071] In another optional implementation manner, by comparing the structural similarity between the cloth sample to be tested and its reconstructed image, the cloth defect is detected according to the degree of similarity. If the difference between the cloth sample to be tested and its reconstructed image is less than the preset difference value, the cloth sample to be tested has no defects; otherwise, the cloth sample to be tested is defective, and the area on the cloth sample to be tested is different from its reconstructed image is the defect location.
[0072] In order to further illustrate the performance of the cloth defect detection method provided by the present invention, the convolutional denoising autoencoder model (CDAE), the multi-scale convolutional denoising autoencoder model (MSCDAE), the U-shaped convolutional denoising autoencoder The encoder model (UCDAE) and the lightweight generative adversarial network (LCD-GAN) proposed by the present invention are trai...
Embodiment 2
[0082] A cloth defect detection system, comprising:
[0083] The image reconstruction module is used to input the cloth sample to be tested into the pre-trained lightweight generative adversarial network to obtain the reconstruction map of the cloth sample to be tested;
[0084] The defect detection module is used to compare the difference between the cloth sample to be tested and its reconstructed image to detect cloth defects;
[0085] The above lightweight generative adversarial network includes a lightweight generator and a discriminator; the lightweight generator is used to generate a reconstructed image of the input cloth sample; the discriminator is used to determine whether the input image is a real cloth sample or a generated reconstructed image ;
[0086] Among them, the lightweight generator includes: encoder and decoder;
[0087] The encoder includes a convolutional layer and a bottleneck module connected in series; the bottleneck module includes a plurality of bot...
PUM
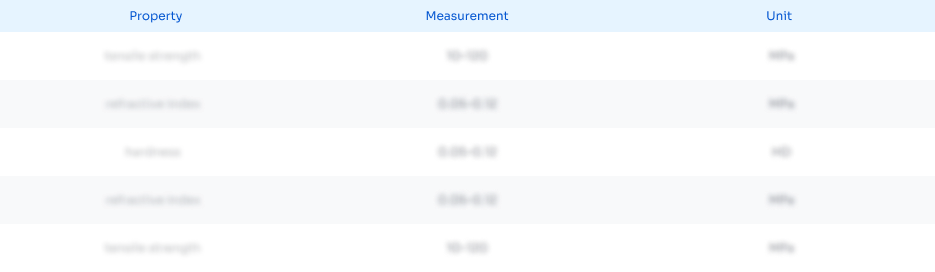
Abstract
Description
Claims
Application Information
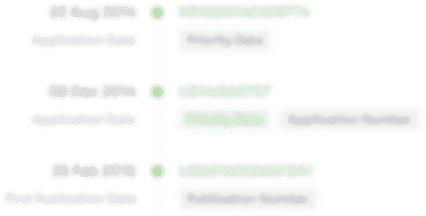
- R&D Engineer
- R&D Manager
- IP Professional
- Industry Leading Data Capabilities
- Powerful AI technology
- Patent DNA Extraction
Browse by: Latest US Patents, China's latest patents, Technical Efficacy Thesaurus, Application Domain, Technology Topic, Popular Technical Reports.
© 2024 PatSnap. All rights reserved.Legal|Privacy policy|Modern Slavery Act Transparency Statement|Sitemap|About US| Contact US: help@patsnap.com