Short-term load prediction method based on long short-term memory network
A technology of short-term load forecasting and long-term short-term memory, applied in forecasting, neural learning methods, biological neural network models, etc., can solve problems such as poor results
- Summary
- Abstract
- Description
- Claims
- Application Information
AI Technical Summary
Problems solved by technology
Method used
Image
Examples
Embodiment Construction
[0043] In order to make the objectives, technical solutions and advantages of the present invention clearer, the present invention will be further described in detail below with reference to the accompanying drawings and embodiments. It should be understood that the specific embodiments described herein are only used to explain the present invention, but not to limit the present invention.
[0044] This application presents as figure 1 A short-term load forecasting method based on long short-term memory network is shown, which includes the following steps:
[0045] S1. Collect short-term load data. In this application, the short-term load data in a certain month in a certain region is obtained by collecting every 15 minutes, such as image 3 shown.
[0046] Take the collected short-term load data as training samples, and perform VMD decomposition (variational mode decomposition) on the short-term load data (training samples) to obtain the random component IMF. After decompo...
PUM
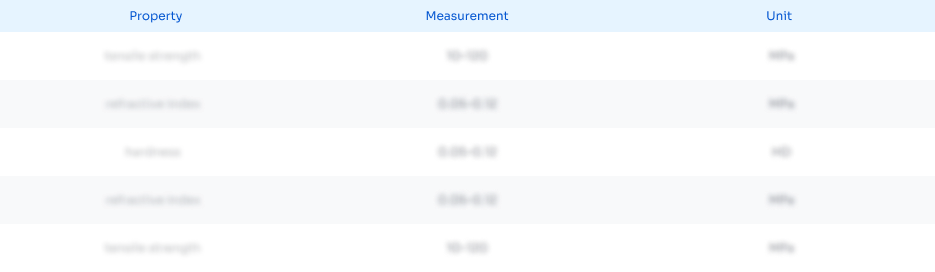
Abstract
Description
Claims
Application Information
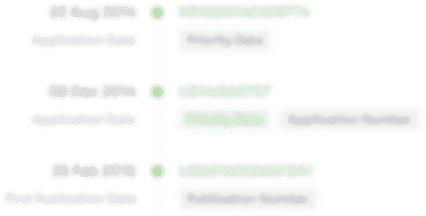
- Generate Ideas
- Intellectual Property
- Life Sciences
- Materials
- Tech Scout
- Unparalleled Data Quality
- Higher Quality Content
- 60% Fewer Hallucinations
Browse by: Latest US Patents, China's latest patents, Technical Efficacy Thesaurus, Application Domain, Technology Topic, Popular Technical Reports.
© 2025 PatSnap. All rights reserved.Legal|Privacy policy|Modern Slavery Act Transparency Statement|Sitemap|About US| Contact US: help@patsnap.com