Industrial control anomaly detection method for industrial control system with insufficient samples
An industrial control system and anomaly detection technology, applied in the direction of comprehensive factory control, instrumentation, calculation, etc., can solve the problems of limited calculation amount, large difference between source domain and target domain, and difficult to obtain
- Summary
- Abstract
- Description
- Claims
- Application Information
AI Technical Summary
Problems solved by technology
Method used
Image
Examples
Embodiment 1
[0064] This experiment runs on Windows10 system, CPU is AMD Ryzen 7 4800H, GPU is NVIDIA GeForceRTX 2060, memory 16.0 GB, Python version 3.7, TensorFlow version 1.14.
[0065] This experiment uses two data sets for experimental verification, one of which is a public data set in the industrial control field as the source domain data, and the other is a self-built data set in the industrial control field as the target domain data. The public data set is the C-Town water distribution data set (referred to as the Singapore water plant data set) constructed by the Cyber Security Research Center of the Singapore University of Science and Technology in 2018 (Riccardo Taormina et al. Battle of the Attack Detection Algorithms: Disclosing Cyber Attacks on Water Distribution Networks[J]. Journal of WaterResources Planning and Management, 2018, 144(8). DOI: 10.1061 / (ASCE)WR.1943-5452.0000969). The Singapore Water Works dataset network pipeline consists of 429 pipes, 388 connection point...
PUM
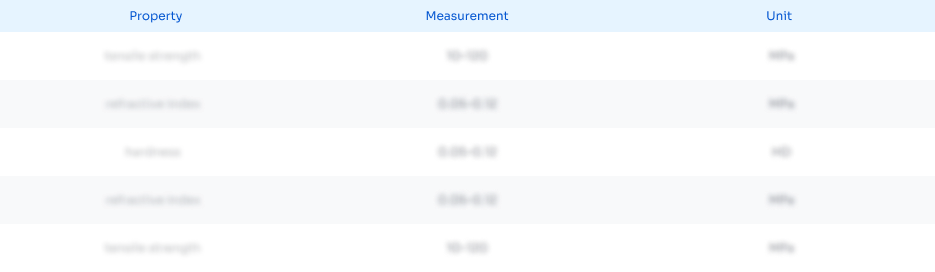
Abstract
Description
Claims
Application Information
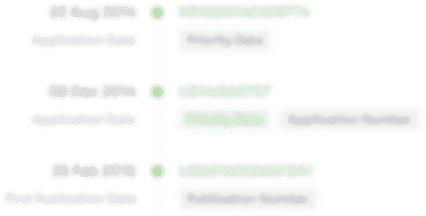
- Generate Ideas
- Intellectual Property
- Life Sciences
- Materials
- Tech Scout
- Unparalleled Data Quality
- Higher Quality Content
- 60% Fewer Hallucinations
Browse by: Latest US Patents, China's latest patents, Technical Efficacy Thesaurus, Application Domain, Technology Topic, Popular Technical Reports.
© 2025 PatSnap. All rights reserved.Legal|Privacy policy|Modern Slavery Act Transparency Statement|Sitemap|About US| Contact US: help@patsnap.com