Deep medical image clustering method based on multi-scale structure learning
A medical image and clustering method technology, applied in the field of deep learning and image clustering, can solve problems such as difficult and complex image representation learning and clustering, multi-scale neighborhood information is not considered, and single-scale neighborhood structure cannot be broken through , to achieve excellent anti-interference ability, improve robustness, and improve confidence
- Summary
- Abstract
- Description
- Claims
- Application Information
AI Technical Summary
Problems solved by technology
Method used
Image
Examples
Embodiment Construction
[0085] Existing deep image clustering methods mainly consider the feature information of the image data itself; some methods consider the fixed neighborhood information between image data, but fail to break through the single-scale neighborhood structure, and also fail to utilize graph convolution. The graph neural network including the network performs multi-scale neighborhood structure learning between image data. How to combine the representation learning of the image itself and the neighborhood structure learning between images based on the deep learning framework, and extend the single-scale neighborhood structure information to the multi-scale neighborhood structure learning, so as to design a more effective deep image clustering method , there is currently no solution.
[0086] The purpose of the present invention is to overcome the shortcomings and deficiencies of the existing deep image clustering methods in multi-scale neighborhood structure learning, and provide a d...
PUM
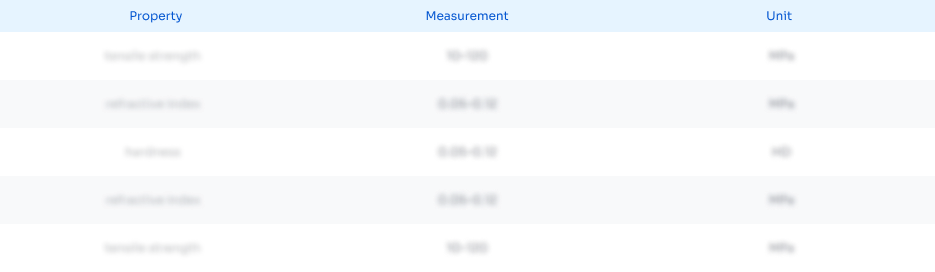
Abstract
Description
Claims
Application Information
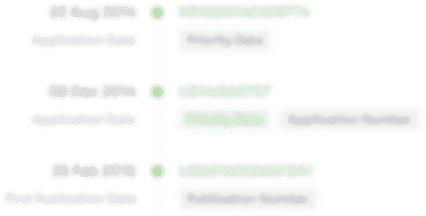
- Generate Ideas
- Intellectual Property
- Life Sciences
- Materials
- Tech Scout
- Unparalleled Data Quality
- Higher Quality Content
- 60% Fewer Hallucinations
Browse by: Latest US Patents, China's latest patents, Technical Efficacy Thesaurus, Application Domain, Technology Topic, Popular Technical Reports.
© 2025 PatSnap. All rights reserved.Legal|Privacy policy|Modern Slavery Act Transparency Statement|Sitemap|About US| Contact US: help@patsnap.com