Electroencephalogram signal classification method based on collaborative contrast regularization average teacher model
A technology of EEG signal and classification method, applied in the field of EEG signal recognition, can solve the problems of ignoring the changing factors of EEG data, difficult to break through the performance bottleneck of EEG recognition model, low quality of EEG data, etc., and achieves enhanced robustness and generalization. Ability, the effect of enhancing discriminative learning ability
- Summary
- Abstract
- Description
- Claims
- Application Information
AI Technical Summary
Problems solved by technology
Method used
Image
Examples
Embodiment Construction
[0024] see figure 1 shown:
[0025] The present invention will be further explained below in conjunction with examples.
[0026] The main implementation process of the present invention is as follows, and the relevant network framework sees figure 1 .
[0027] Step 1: Collect EEG data.
[0028] Step 2: Preprocess the EEG data, including band-pass filtering and artifact removal, select specific time periods and frequency bands, and obtain EEG data in Represents the nth EEG data, c, d represent the number of electrode channels and time sampling points of EEG data, respectively, y n ∈{0, 1, 2, 3} is the label corresponding to the nth EEG data.
[0029] Step 3: Segment and reorganize the EEG data according to two different data augmentation methods, and obtain the augmented EEG data from different perspectives and The specific EEG data augmentation methods are as follows:
[0030] 1) Use short-time Fourier transform on the preprocessed EEG data, and convert it from th...
PUM
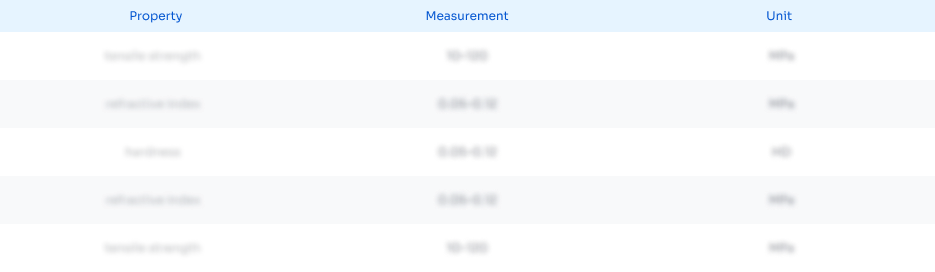
Abstract
Description
Claims
Application Information
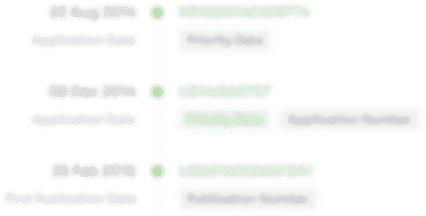
- R&D Engineer
- R&D Manager
- IP Professional
- Industry Leading Data Capabilities
- Powerful AI technology
- Patent DNA Extraction
Browse by: Latest US Patents, China's latest patents, Technical Efficacy Thesaurus, Application Domain, Technology Topic, Popular Technical Reports.
© 2024 PatSnap. All rights reserved.Legal|Privacy policy|Modern Slavery Act Transparency Statement|Sitemap|About US| Contact US: help@patsnap.com