Semi-supervised medical image classification method based on security comparison self-integration framework
A medical image and classification method technology, applied in the field of medical image classification, can solve the problems of ignoring the reliability, security and generalization limitations of unlabeled medical images, and achieve good intra-class compactness and inter-class separability, Improves security and generalization, and mitigates performance degradation
- Summary
- Abstract
- Description
- Claims
- Application Information
AI Technical Summary
Problems solved by technology
Method used
Image
Examples
Embodiment Construction
[0025] see figure 1 shown:
[0026] The present invention will be further explained below in conjunction with examples.
[0027] The main implementation process of the present invention is as follows, and the relevant network framework sees figure 1 .
[0028] Step 1: Divide the image into labeled data with untagged data where x i represents the ith medical image sample, y i represents the true label of the ith sample;
[0029] Step 2: Take a batch of training data consisting of unlabeled samples and labeled samples, and perform random rotation and affine transformation to increase data disturbance. degrees, 10 degrees), and the corresponding translation interval parameters of the length and width dimensions are (0.02, 0.02). After repeating the processing twice, two groups of input data with increased disturbance are obtained, and the two groups of data are input into the student network and the teacher network of the average teacher model respectively.
[0030] St...
PUM
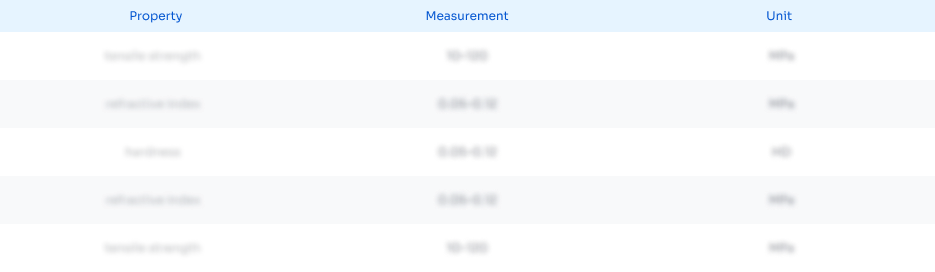
Abstract
Description
Claims
Application Information
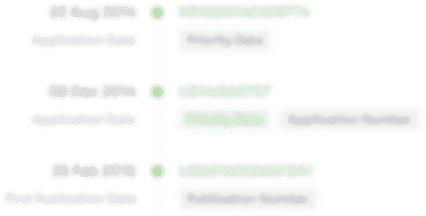
- R&D Engineer
- R&D Manager
- IP Professional
- Industry Leading Data Capabilities
- Powerful AI technology
- Patent DNA Extraction
Browse by: Latest US Patents, China's latest patents, Technical Efficacy Thesaurus, Application Domain, Technology Topic, Popular Technical Reports.
© 2024 PatSnap. All rights reserved.Legal|Privacy policy|Modern Slavery Act Transparency Statement|Sitemap|About US| Contact US: help@patsnap.com