Carrier image enhancement method based on generative network
A carrier image, generative technology, applied in the field of information hiding, which can solve the problems of weak mobility, low security, and deceiving non-target steganalyzers.
- Summary
- Abstract
- Description
- Claims
- Application Information
AI Technical Summary
Problems solved by technology
Method used
Image
Examples
Embodiment 1
[0028] A carrier image enhancement method based on a generative network. By adding anti-noise to the carrier image, an enhanced carrier image is obtained. The enhanced carrier image can effectively deceive the steganalyzer and the traditional steganalysis method SRM after embedding secret information.
[0029] A. Training phase (eg figure 1 shown)
[0030] Step 1. Input the carrier image c in the training set into the anti-noise generator G. The anti-noise generator G can choose a fully convolutional network such as FCN and U-Net. In this example, the generator selects the FCN network whose structure is as follows figure 2 shown. The anti-noise generator will obtain the anti-noise n according to the content information of the carrier image.
[0031] Step 2. Add the adversarial noise n to the carrier image c to obtain the enhanced adversarial image adv_c.
[0032] Step 3. Use S-UNIWARD as the adaptive steganography algorithm in this example, generate steganographic noise s...
Embodiment 2
[0049] In order to improve the mobility, the present invention also considers a mobility improvement strategy based on a dual-target steganalyzer, according to the differences in the mobility of the anti-noise generators obtained when training with different target steganalyzers. Divide the steganalyzers into two groups, and then extract a steganalyzer from the two groups to combine, and jointly train as the target steganalyzer to provide adversarial loss, and the rest is the same as when attacking a single steganalyzer. keep it the same. Using two different steganalysis networks as the target steganalyzer can help the adversarial noise generator better learn the differences between the two different steganalyzers and find the common weaknesses between the two models, Generate more mobile adversarial noise.
[0050] A. Training phase (eg image 3 shown)
[0051] Specifically, steps 1 to 6 are the same as those in Embodiment 1.
[0052] Step 7. When implementing the mobilit...
PUM
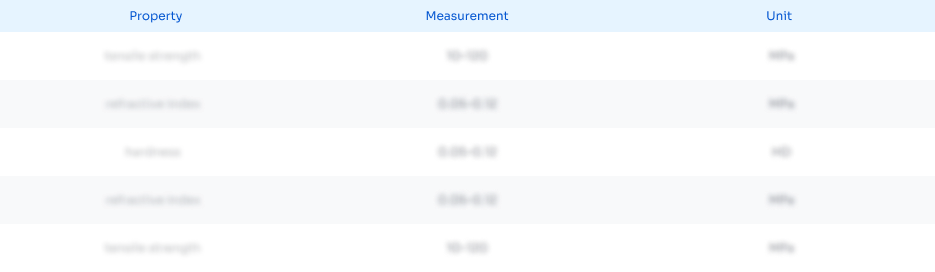
Abstract
Description
Claims
Application Information
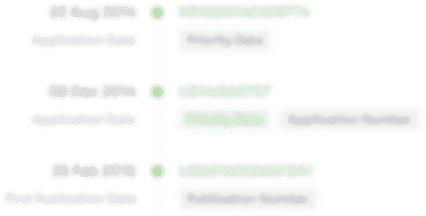
- R&D Engineer
- R&D Manager
- IP Professional
- Industry Leading Data Capabilities
- Powerful AI technology
- Patent DNA Extraction
Browse by: Latest US Patents, China's latest patents, Technical Efficacy Thesaurus, Application Domain, Technology Topic, Popular Technical Reports.
© 2024 PatSnap. All rights reserved.Legal|Privacy policy|Modern Slavery Act Transparency Statement|Sitemap|About US| Contact US: help@patsnap.com