Human motion behavior recognition method
A technology of human motion and recognition methods, applied in the field of motion recognition, can solve problems such as the skeleton structure and influence of the human body that cannot be well represented, and achieve the effect of reducing data storage and computing overhead
- Summary
- Abstract
- Description
- Claims
- Application Information
AI Technical Summary
Problems solved by technology
Method used
Image
Examples
specific Embodiment approach 1
[0014] Specific implementation one: as figure 1 As shown, a method for recognizing human motion behavior described in this embodiment includes the following steps:
[0015] Step 1. Use the motion capture system to collect data on multiple movements of the human body, and use 9-axis sensors for all joints of the human body when collecting the data of each movement, such as figure 2 As shown, the 9-axis sensor includes a 3-axis accelerometer, a 3-axis gyroscope, and a 3-axis magnetometer, and the acquisition includes the three-axis accelerometer data, the three-axis gyroscope data, the three-axis magnetometer data, and the three-axis attitude angle. the raw data of the data;
[0016] Step 2: Construct a CRF conditional random field model applied to text sequence data lambda=CRF(w1,w2,...,wn), where w1 to wn are model parameters;
[0017] Step 3: Convert the time series of human motion raw data including all joints into feature vectors, mark the corresponding motion types, and...
specific Embodiment approach 2
[0020] Embodiment 2: In Embodiment 2, a fixed number of implicit sequences are defined. The CRF model is trained by using the time series feature vector, latent sequence and human motion behavior type of human motion raw data as corpus.
[0021] A fixed number of implicit sequences are defined, and semantic features of human motion behavior are assigned to the implicit sequences, which can greatly reduce the number of elements in the implicit sequences and improve the training efficiency.
[0022] For example, a latent sequence can be defined based on how much each joint changes between two frames. Suppose 10 joints are selected, and the 60-degree variation range of each joint in the space X plane, Y plane and Z plane is used as an element of the implicit sequence, which can be defined to include 3 3 *10 = implicit sequence of 270 elements.
[0023] Implicit sequences can also be defined based on the direction of motion of each joint. Assuming that 10 joints are selected, t...
PUM
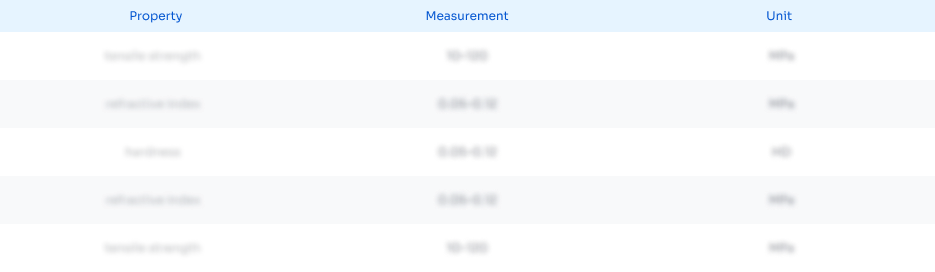
Abstract
Description
Claims
Application Information
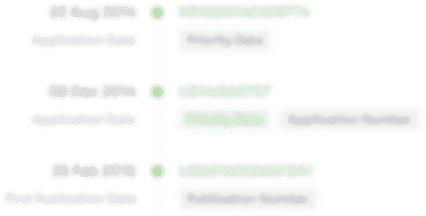
- R&D Engineer
- R&D Manager
- IP Professional
- Industry Leading Data Capabilities
- Powerful AI technology
- Patent DNA Extraction
Browse by: Latest US Patents, China's latest patents, Technical Efficacy Thesaurus, Application Domain, Technology Topic, Popular Technical Reports.
© 2024 PatSnap. All rights reserved.Legal|Privacy policy|Modern Slavery Act Transparency Statement|Sitemap|About US| Contact US: help@patsnap.com