Using deep learning based defect detection and classification schemes for pixel level image quantification
a deep learning and defect detection technology, applied in image enhancement, instruments, image data processing, etc., can solve the problems of affecting the electrical parameters of the device, the operation of the semiconductor manufacturing process closer to the limitation of the performance capability of the process, and the failure to detect defects of decreasing siz
- Summary
- Abstract
- Description
- Claims
- Application Information
AI Technical Summary
Benefits of technology
Problems solved by technology
Method used
Image
Examples
Embodiment Construction
[0042]Although claimed subject matter will be described in terms of certain embodiments, other embodiments, including embodiments that do not provide all of the benefits and features set forth herein, are also within the scope of this disclosure. Various structural, logical, process step, and electronic changes may be made without departing from the scope of the disclosure. Accordingly, the scope of the disclosure is defined only by reference to the appended claims.
[0043]Embodiments disclosed herein use deep learning-based defect detection and / or classification networks for pixel level image quantification. For example, deep learning can be used for pixel level quantification of SEM images. This can be used in applications like extreme ultraviolet (EUV) stochastics rate quantification or critical dimension (CD) measurements / comparison. Embodiments disclosed herein can be integrated in the existing defect detection or classification schemes for review tools or inspection tools.
[0044]...
PUM
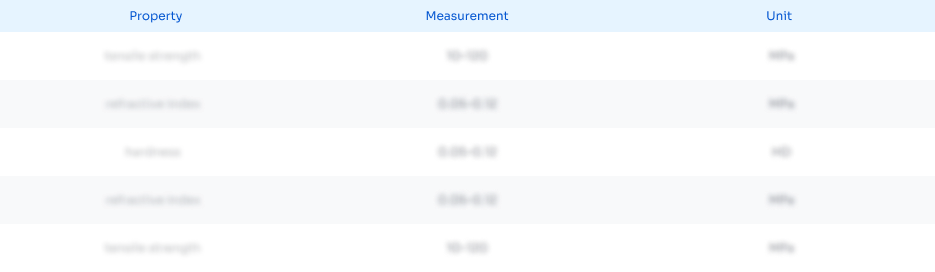
Abstract
Description
Claims
Application Information
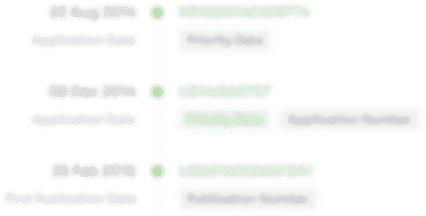
- R&D
- Intellectual Property
- Life Sciences
- Materials
- Tech Scout
- Unparalleled Data Quality
- Higher Quality Content
- 60% Fewer Hallucinations
Browse by: Latest US Patents, China's latest patents, Technical Efficacy Thesaurus, Application Domain, Technology Topic, Popular Technical Reports.
© 2025 PatSnap. All rights reserved.Legal|Privacy policy|Modern Slavery Act Transparency Statement|Sitemap|About US| Contact US: help@patsnap.com