Periodic associated rule discovery algorithm based on time sequence vector diverse sequence method clustering
A technology of difference sequences and timing vectors, applied in computing, special data processing applications, instruments, etc., can solve problems such as time-consuming, large candidate item sets, and low operating efficiency
- Summary
- Abstract
- Description
- Claims
- Application Information
AI Technical Summary
Problems solved by technology
Method used
Image
Examples
Embodiment Construction
[0021] (1) Related basic concepts and properties of time domain data
[0022] Definition 1 (time-domain data). Time-domain data refers to transaction sets with time attributes. Let the time zone of the entire transaction set be T, which can be expressed as T=∪T i ;T i ∩ T j = 0; |T j |=|T i |Where i≠j; i, j=1, 2,..., n|T i | means T i length of time. here called |T i |is a cycle length, T i is the i-th cycle, |T i |Length is user defined such as 1 year, 1 month or 1 week. The goal of the invention is to find at all periods T i The association relationship between some frequent items in a certain period of time.
[0023] Definition 2 (time series vector). A series of observations obtained in time order, each observation value is an n-dimensional vector, these vectors with time attributes are called time series vectors.
[0024] The time series vector sequence composed of time series vectors is a time series (see the text for related concepts of time series [17] )...
PUM
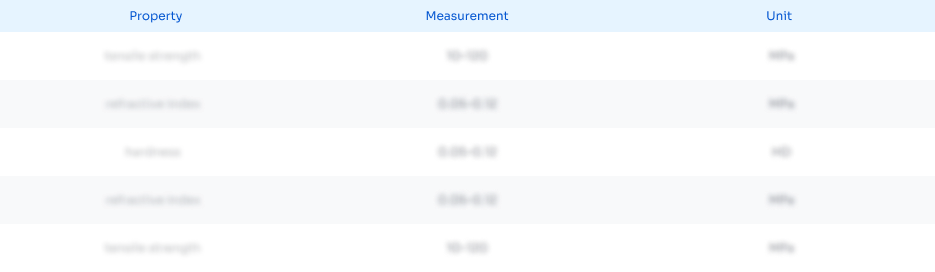
Abstract
Description
Claims
Application Information
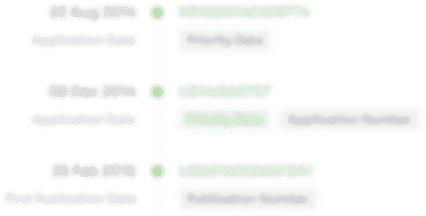
- R&D
- Intellectual Property
- Life Sciences
- Materials
- Tech Scout
- Unparalleled Data Quality
- Higher Quality Content
- 60% Fewer Hallucinations
Browse by: Latest US Patents, China's latest patents, Technical Efficacy Thesaurus, Application Domain, Technology Topic, Popular Technical Reports.
© 2025 PatSnap. All rights reserved.Legal|Privacy policy|Modern Slavery Act Transparency Statement|Sitemap|About US| Contact US: help@patsnap.com