Hardware circuit of recursive neural network of LS-SVM classification and returning study and implementing method
A recurrent neural network, LS-SVM technology, applied in the field of pattern recognition
- Summary
- Abstract
- Description
- Claims
- Application Information
AI Technical Summary
Problems solved by technology
Method used
Image
Examples
example 1
[0113] z 1 =(1.5,2),z 2 =(2,1),z 3 =(1.5,1),z 4 =(3,4),z 5 =(4, 3), and its categories are (+1, +1, -1, -1, -1) respectively.
[0114] Step 1: Construct the topology structure of the SVM classification learning recurrent neural network according to the number of 5 samples;
[0115] Step 2: Use Gaussian kernel function, choose σ=1.5, γ -1 = 0.20, and calculate
[0116] Step 3: select corresponding module according to classification neurorecurrent network topology and carry out Simulink simulation based on Matlab software;
[0117] Step 4: Select R 0 =1kΩ, C=1μF, calculate the resistance R of each weight 0 / |q ij |, and use the "rounding" method to select the nominal resistance value as close as possible;
[0118] Step 5: According to figure 2 The PCB hardware circuit is made according to the structure, in which the package of the resistor is AXIAL0.4, the package of the operational amplifier is DIP8, the package of the capacitor is RB.2 / .4, and the integral part i...
example 2
[0129] Table 2 Function values of 9 points
[0130]
[0131] Step 1: Construct the topology structure of the SVM regression neural network according to the number of 9 samples;
[0132] Step 2: Use Gaussian kernel function, where σ=1, select γ -1 =0.01, and calculate Ω according to the sample points ij =K(x i , x j )=φ(x i ) T φ(x j );
[0133] Step 3: Select the corresponding module according to the topology of the regression neural network to carry out Simulink simulation based on Matlab software;
[0134] Step 4: Select R 0 =1kΩ, C=1μF, calculate the resistance R of each weight 0 / |Ω ij |, and use the "rounding" method to select the nominal resistance value as close as possible;
[0135] Step 5: Due to the resistance value R 0 / |Ω ij |It is very large in theory, close to the MΩ level, so the correctness of the analog circuit is verified in the form of Pspice simulation. The operational amplifier used in the analog implementation circuit is μA741, and all ...
PUM
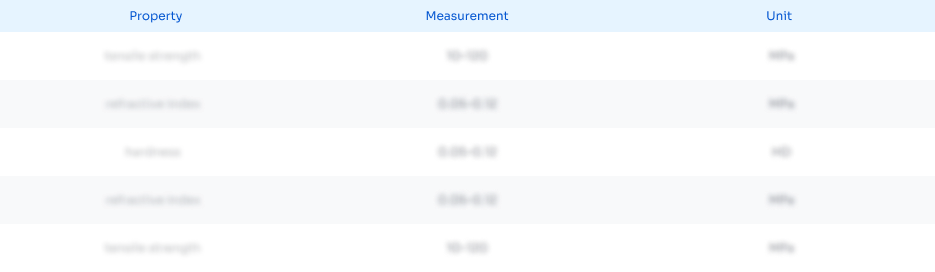
Abstract
Description
Claims
Application Information
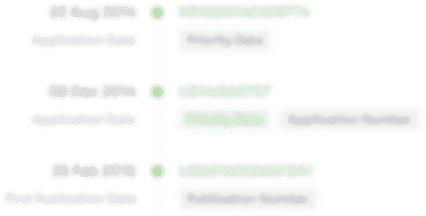
- R&D Engineer
- R&D Manager
- IP Professional
- Industry Leading Data Capabilities
- Powerful AI technology
- Patent DNA Extraction
Browse by: Latest US Patents, China's latest patents, Technical Efficacy Thesaurus, Application Domain, Technology Topic, Popular Technical Reports.
© 2024 PatSnap. All rights reserved.Legal|Privacy policy|Modern Slavery Act Transparency Statement|Sitemap|About US| Contact US: help@patsnap.com