Formulae neighborhood based data dimensionality reduction method
A data dimensionality reduction and neighborhood technology, applied in the field of information processing, can solve the problems of being too sensitive to parameters and external noise, and the failure of dimensionality reduction performance, so as to achieve the effect of widening the applicable neighborhood, maintaining consistency, and improving the aggregation effect.
- Summary
- Abstract
- Description
- Claims
- Application Information
AI Technical Summary
Problems solved by technology
Method used
Image
Examples
Embodiment Construction
[0015] As shown in the attached figure, a data dimensionality reduction method based on rule neighborhood includes the following contents:
[0016] 1. Formal description of data dimensionality reduction
[0017] Establish a quintuple model: FO=(X, D, δ, d, Y),
[0018] Among them: D is the dimension of the high-dimensional space; d (d X = { x → 1 , x → 2 , . . . , x → N } , is the high-dimensional space R D N D-dimensional real vectors in ( x → i = ( x → i 1 ...
PUM
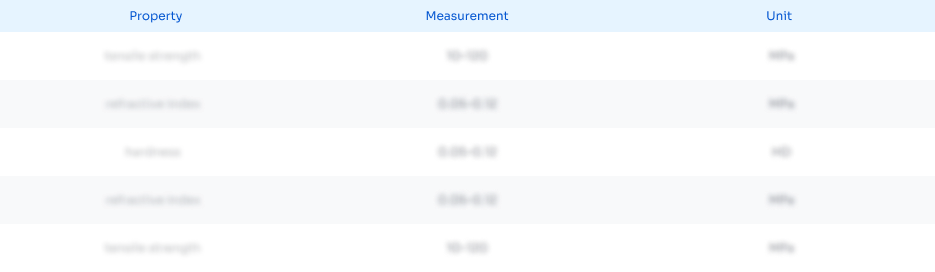
Abstract
Description
Claims
Application Information
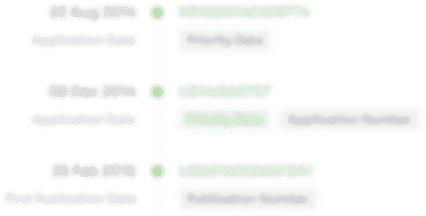
- R&D Engineer
- R&D Manager
- IP Professional
- Industry Leading Data Capabilities
- Powerful AI technology
- Patent DNA Extraction
Browse by: Latest US Patents, China's latest patents, Technical Efficacy Thesaurus, Application Domain, Technology Topic, Popular Technical Reports.
© 2024 PatSnap. All rights reserved.Legal|Privacy policy|Modern Slavery Act Transparency Statement|Sitemap|About US| Contact US: help@patsnap.com