Infrared image background suppression method based on unsupervised kernel regression analysis
An infrared image and background suppression technology, applied in the field of infrared image background suppression, can solve problems such as requiring prior knowledge and poor adaptability, and achieve good nonlinear data prediction ability, good background effect, and good local adaptive prediction ability. Effect
- Summary
- Abstract
- Description
- Claims
- Application Information
AI Technical Summary
Problems solved by technology
Method used
Image
Examples
specific Embodiment approach 1
[0018] Specific implementation mode one: combine figure 1 Describe this embodiment, the steps of this embodiment are as follows:
[0019] Step 1. Set the sliding window for background prediction; the sliding window adopts a double-window mode to predict the background clutter, and the sliding window is composed of inner window B and outer window A; inner window B is located at the center of the sliding window, and inner window B’s The center is the center test sample O, and the inner window B is used to protect the information of the center test sample O located in the center of the sliding window. The area of the inner window B outside the center test sample O is equivalent to the protection area to prevent the test sample O from being the target pixel. In the process of selecting background clutter samples, the samples related to the target are selected, so the protection area is generated; the outside of the inner window B of the sliding window is the outer window A, and ...
specific Embodiment approach 2
[0025] Specific implementation mode two: combination figure 2 This embodiment is described. The first difference between this embodiment and the specific embodiment is that if the central test sample O of the inner window B currently being processed is located at the edge of the infrared image C, the samples of the missing part in the sliding window are obtained by mirror symmetry. , that is to use mirror symmetry to obtain the gray value of missing pixels. Other steps are the same as in the first embodiment.
specific Embodiment approach 3
[0026] Specific embodiment three: the difference between this embodiment and specific embodiment one is that the steps for obtaining the unsupervised kernel regression equation in step three are as follows:
[0027] The regression estimation formula is as follows:
[0028] the y i =z(x i )+ε i , i=1, ..., P, (1)
[0029] where x i It is a 2x1-dimensional vector, representing the coordinates of the two-dimensional space, y i Represents the corresponding image gray value; z(x i ) is called the regression function, ε i For random error or random disturbance, it is a distribution with x i An irrelevant random variable, which is a normally distributed random variable with a mean of 0; the z(x i ) is expanded in the neighborhood, the following formula can be obtained:
[0030] z(x i )=β 0 +β 1 T (x i -x)+β 2 T vech{(x i -x)(x i -x) T}+... (2)
[0031] The definition of vech( ) is the vectorization of the lower triangular part of the symmetric matrix, with a 2×2 s...
PUM
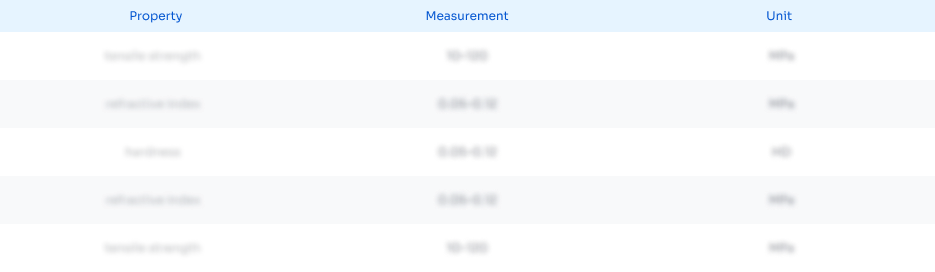
Abstract
Description
Claims
Application Information
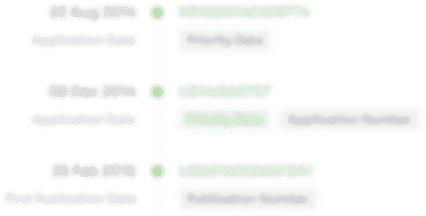
- R&D Engineer
- R&D Manager
- IP Professional
- Industry Leading Data Capabilities
- Powerful AI technology
- Patent DNA Extraction
Browse by: Latest US Patents, China's latest patents, Technical Efficacy Thesaurus, Application Domain, Technology Topic, Popular Technical Reports.
© 2024 PatSnap. All rights reserved.Legal|Privacy policy|Modern Slavery Act Transparency Statement|Sitemap|About US| Contact US: help@patsnap.com