Nonnegative matrix factorization-based dimensionality reducing method used for clustering
A non-negative matrix factorization and clustering technology, applied in the field of dimensionality reduction based on non-negative matrix factorization, it can solve the problems of dimensional disaster, multi-computing time, high storage and computing costs, and achieve the effect of good sparsity
- Summary
- Abstract
- Description
- Claims
- Application Information
AI Technical Summary
Problems solved by technology
Method used
Image
Examples
Embodiment 1
[0053] Embodiment 1 can more intuitively illustrate the characteristics of the matrices X, C and M. matrix
[0054] A=20001032100232020002
[0055] It is a simple data matrix. For text data, each column corresponds to a different word, and each row represents a statistical vector of the number of occurrences of different words in a certain text. Our task is to group similar texts together in a compressed low-dimensional space. In order to simplify the above problem, the data in data matrix A can be binarized first, that is,
[0056] A ij = 1 if A ij > 0 0 else
[0057] Based on this, NCMF can be decomposed as follows,
[0058] 1 / 20001 / 201 / 21 / 21 / 2001 / 21 / 21 / 201 / 20001 / 2=1 / 2001 / 201 / 21 / 201000101110---(8)
[0059] When the three matrices corresponding to the above decomposition are represented by X, C, and M respectively, X=CM is obtained. The matrix X is composed of 4 sample row vectors, each sample has 5 dimensions (columns), and the...
Embodiment 2
[0060] Example 2 illustrates the experimental results on the gait data set [17]. The original data of this data set is some video data, and the frame data extracted from it to eliminate the background are as attached figure 2 . In order to apply these gait data, the data information can be further extracted to form a data matrix with several sample feature vectors for each ID number (corresponding to a person). In order to illustrate the dimensionality reduction characteristics of the NCMF algorithm, the present invention uses the first 6 ID numbers from the most samples in the galData data to perform cluster analysis on the corresponding data. For the related results, we use three measurement methods, running time, sparsity and clustering accuracy, which are measured in seconds, information entropy, and purity.
[0061] The data after dimensionality reduction is clustered using the same class k-means algorithm SIB [18], and the clustering accuracy is measured by purity. If t...
PUM
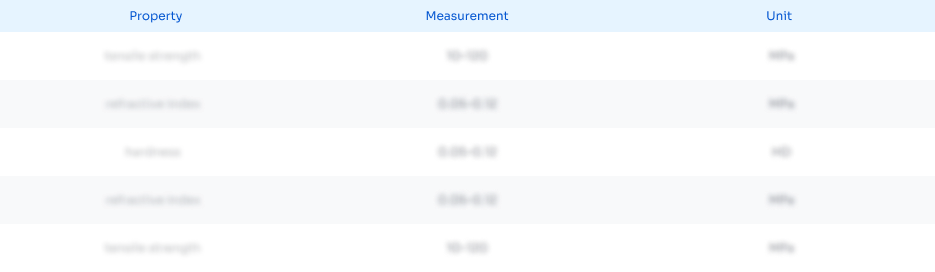
Abstract
Description
Claims
Application Information
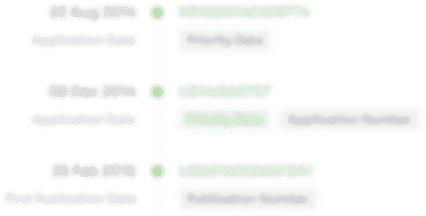
- R&D
- Intellectual Property
- Life Sciences
- Materials
- Tech Scout
- Unparalleled Data Quality
- Higher Quality Content
- 60% Fewer Hallucinations
Browse by: Latest US Patents, China's latest patents, Technical Efficacy Thesaurus, Application Domain, Technology Topic, Popular Technical Reports.
© 2025 PatSnap. All rights reserved.Legal|Privacy policy|Modern Slavery Act Transparency Statement|Sitemap|About US| Contact US: help@patsnap.com