Semi-supervised anomaly intrusion detection method
An intrusion detection and semi-supervisory technology, applied in the field of network security, can solve the problems of low false alarm rate, high false alarm rate, and inability to effectively detect unknown intrusion behaviors, so as to reduce false alarm rate, improve detection rate, and high The effect of detection rate
- Summary
- Abstract
- Description
- Claims
- Application Information
AI Technical Summary
Problems solved by technology
Method used
Image
Examples
Embodiment Construction
[0023] refer to figure 1 , the specific implementation steps of the present invention are as follows:
[0024] Step 1. Select an initial labeled sample set and an initial unlabeled sample set.
[0025] When performing intrusion detection, the detection data corresponding to normal behavior is defined as normal data, the detection data corresponding to various intrusion behaviors is defined as abnormal data, and part of the normal data in the training data is extracted as the initial labeled sample set {x i}, taking the detection data as the initial unlabeled sample set {x j}.
[0026] Step 2, initialize the cluster centers of the detection data.
[0027] Implement the fuzzy C-means algorithm on the current marked and unmarked samples, and repeat the following operation steps until the membership value of the marked and unmarked samples is stable:
[0028] (2a) Calculate membership degree:
[0029] u ck = ...
PUM
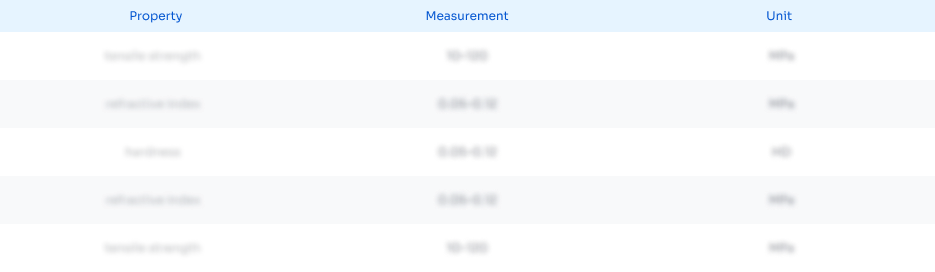
Abstract
Description
Claims
Application Information
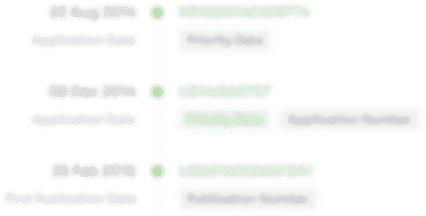
- R&D
- Intellectual Property
- Life Sciences
- Materials
- Tech Scout
- Unparalleled Data Quality
- Higher Quality Content
- 60% Fewer Hallucinations
Browse by: Latest US Patents, China's latest patents, Technical Efficacy Thesaurus, Application Domain, Technology Topic, Popular Technical Reports.
© 2025 PatSnap. All rights reserved.Legal|Privacy policy|Modern Slavery Act Transparency Statement|Sitemap|About US| Contact US: help@patsnap.com