Image classifying method for combining vision vocabulary books of different sizes
A visual vocabulary and classification method technology, applied in the direction of instruments, character and pattern recognition, computer components, etc., can solve the problems of image classification that cannot effectively integrate multiple information, unstable generalization ability, poor model, etc., and achieve low requirements , improve performance, and model simple effects
- Summary
- Abstract
- Description
- Claims
- Application Information
AI Technical Summary
Problems solved by technology
Method used
Examples
specific Embodiment
[0021] A descriptor corresponds to its nearest word in Euler space. After forming a member vocabulary, in order to quantify the image, all detected interest points are used to build a histogram based on this member vocabulary. To make the histogram independent of the number of descriptors, the histogram vector is normalized to sum to 1. The visual vocabulary is obtained by applying a clustering algorithm to a set of 200,000 descriptors randomly selected from the training image set. Weighted LibSVM is used to train the classifier. In the training phase, the weight of positive samples is set to , and the weight of the negative sample is set to , where #pos represents the number of positive samples in the training set, and #neg is the number of negative samples in the training set. In order to apply the SVM (Support Vector Machine) classifier to multi-class problems, a one-against-all method is applied.
[0022] For interest point detectors and descriptors, use the color d...
PUM
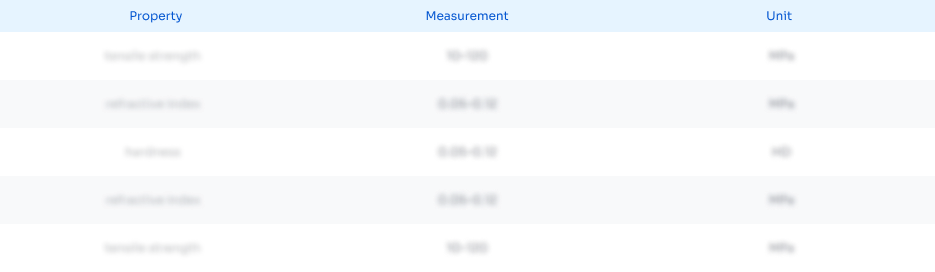
Abstract
Description
Claims
Application Information
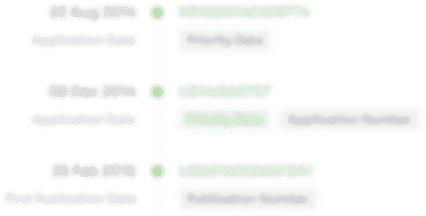
- R&D Engineer
- R&D Manager
- IP Professional
- Industry Leading Data Capabilities
- Powerful AI technology
- Patent DNA Extraction
Browse by: Latest US Patents, China's latest patents, Technical Efficacy Thesaurus, Application Domain, Technology Topic, Popular Technical Reports.
© 2024 PatSnap. All rights reserved.Legal|Privacy policy|Modern Slavery Act Transparency Statement|Sitemap|About US| Contact US: help@patsnap.com