Combination forecast modeling method of wind farm power by using gray correlation analysis
A technology of grey correlation degree and power combination, applied in prediction, neural learning method, data processing application, etc., can solve problems such as difficult to obtain global optimal solution, easy to fall into local minimum, etc.
- Summary
- Abstract
- Description
- Claims
- Application Information
AI Technical Summary
Problems solved by technology
Method used
Image
Examples
Embodiment Construction
[0041] The present invention provides a wind farm power combination prediction modeling method using gray correlation degree analysis. The wind farm power combination prediction modeling method is a weighted combination prediction of wind power based on least squares support vector machine and back propagation neural network Method; The present invention will be described in further detail below in conjunction with accompanying drawing.
[0042] figure 1 It is a structural diagram of the forecasting system of the present invention, illustrating each component and function of the system. The prediction system collects the forecasted values of wind speed and wind direction from the meteorological department in advance, and collects real-time output power from the wind farm data acquisition system (SCADA); input the two into the data processing module for data analysis, extraction and normalization, and then import them into the database server; The combined forecasting algorith...
PUM
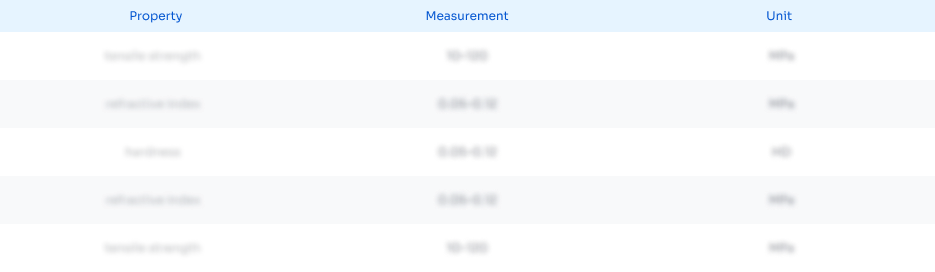
Abstract
Description
Claims
Application Information
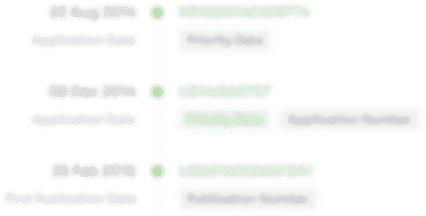
- Generate Ideas
- Intellectual Property
- Life Sciences
- Materials
- Tech Scout
- Unparalleled Data Quality
- Higher Quality Content
- 60% Fewer Hallucinations
Browse by: Latest US Patents, China's latest patents, Technical Efficacy Thesaurus, Application Domain, Technology Topic, Popular Technical Reports.
© 2025 PatSnap. All rights reserved.Legal|Privacy policy|Modern Slavery Act Transparency Statement|Sitemap|About US| Contact US: help@patsnap.com