Self-learning method for improving forecasting precision of overall length coiling temperature of strip steel
A self-learning method and technology of coiling temperature, applied in the field of automatic control of hot-rolled strip, can solve the problems of complex change law, destroying the continuity of self-learning between sections, and restricting practical application, and achieve good on-site application effect and on-site debugging. The effect of flexibility, convenience and strong adaptability
- Summary
- Abstract
- Description
- Claims
- Application Information
AI Technical Summary
Problems solved by technology
Method used
Image
Examples
Embodiment Construction
[0023] The technical solutions of the present invention will be further described below in conjunction with specific embodiments.
[0024] Taking the front and rear strips with a thickness of 13.5mm, a length of 185m, and a steel type of Q345B as an example, the entire length of the strip is divided into about 55 sections during the actual coiling temperature control process. Table 1 lists the collected The distance from each section of the previous piece of steel (rolled piece ID is H111982410) to the strip head position p, and the time to start the coiling temperature forecast τ ff , the time τ when reaching the coiling thermometer CT And the actual value f of the self-learning coefficient back-calculated according to the measured coiling temperature * , where the distance between the strip section and the strip head position p is expressed by the percentage of the strip length.
[0025] Table 1H111982410 Control parameters of each section of steel strip
[0026]
[00...
PUM
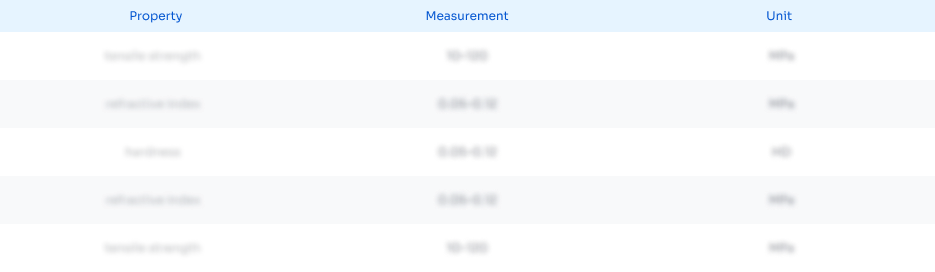
Abstract
Description
Claims
Application Information
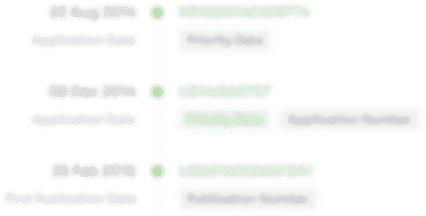
- Generate Ideas
- Intellectual Property
- Life Sciences
- Materials
- Tech Scout
- Unparalleled Data Quality
- Higher Quality Content
- 60% Fewer Hallucinations
Browse by: Latest US Patents, China's latest patents, Technical Efficacy Thesaurus, Application Domain, Technology Topic, Popular Technical Reports.
© 2025 PatSnap. All rights reserved.Legal|Privacy policy|Modern Slavery Act Transparency Statement|Sitemap|About US| Contact US: help@patsnap.com