Hyperspectral image classification method based on F-EEMD (fast ensemble empirical mode decomposition)
A technology that integrates empirical modalities and hyperspectral images, applied in the field of remote sensing, can solve the problems of hyperspectral image preprocessing, such as long preprocessing time, noise addition, large decomposition times, and large computational load, so as to maintain superior performance, improve accuracy and classify Speed, the effect of reducing the number of support vectors
- Summary
- Abstract
- Description
- Claims
- Application Information
AI Technical Summary
Problems solved by technology
Method used
Image
Examples
specific Embodiment approach 1
[0043] Specific implementation mode one: as figure 1 As shown, the specific steps of the hyperspectral image classification algorithm method based on fast ensemble empirical mode decomposition in this embodiment are as follows:
[0044] Step 1: Analyze the influence of IMF screening times K and noise addition and decomposition times N on ensemble empirical mode decomposition.
[0045] This step first graphically analyzes the influence of different IMF screening times K and different noise adding and decomposition times N on the empirical mode decomposition results of the hyperspectral image set. Then, mutual information is used to analyze the influence of IMF screening times N and EEMD noise addition and decomposition times N on EEMD results.
[0046] Step 2: Propose a fast ensemble empirical mode decomposition algorithm (F-EEMD) based on the mutual information threshold, and use the fast ensemble empirical mode decomposition algorithm to perform feature extraction and featur...
specific Embodiment approach 2
[0064] Specific embodiment two: set forth the specific embodiment of the present invention below by the classification example of the hyperspectral data 92AV3C that AVIRIS sensor gathers in Northwestern Indiana, U.S.A.:
[0065] Execution step 1: The influence of the number of IMF screening K and the number of noise addition and decomposition N on the set empirical mode decomposition.
[0066] The influence of IMF screening times K and noise addition and decomposition times N on the ensemble empirical mode decomposition is as follows:
[0067] 1). The influence of IMF screening times K on the results of ensemble empirical mode decomposition: the spatial information of the 20th band is selected as the research object. Carry out ensemble empirical mode decomposition under different IMF screening times K for spatial information, such as Figure 3-10 shown. Among them, H3(k) represents IMF3 when the number of screening is k. It can be seen from the image changes that the intern...
PUM
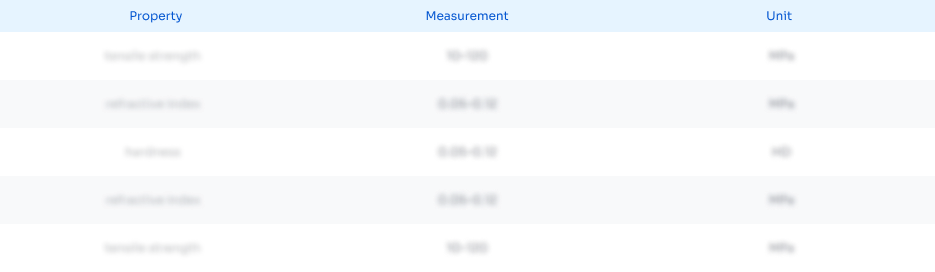
Abstract
Description
Claims
Application Information
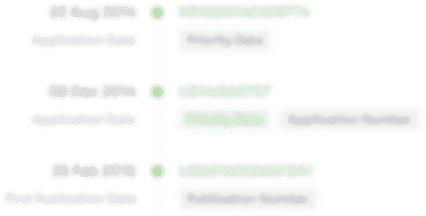
- R&D
- Intellectual Property
- Life Sciences
- Materials
- Tech Scout
- Unparalleled Data Quality
- Higher Quality Content
- 60% Fewer Hallucinations
Browse by: Latest US Patents, China's latest patents, Technical Efficacy Thesaurus, Application Domain, Technology Topic, Popular Technical Reports.
© 2025 PatSnap. All rights reserved.Legal|Privacy policy|Modern Slavery Act Transparency Statement|Sitemap|About US| Contact US: help@patsnap.com