No-reference image quality evaluation method based on Curvelet transformation and phase coincidence
A quality evaluation and reference image technology, applied in the field of image analysis, can solve the problems of long time-consuming feature dimension classification and regression model training, long time-consuming feature extraction process, and large time complexity
- Summary
- Abstract
- Description
- Claims
- Application Information
AI Technical Summary
Problems solved by technology
Method used
Image
Examples
Embodiment 1
[0095] We use the most popular LIVE image quality database to run CPNR to evaluate the performance of the algorithm, divide the entire database into two parts, the training set and the test set, and use the 5-fold cross-validation to select the optimal training parameters, and use the training set to train and build The classifier and regression model required by the algorithm, and then run the test set to evaluate the performance of the algorithm. In order to improve the effectiveness of the results, we follow the following rules when dividing the training and test sets: 1) The training set and the test set are absolutely isolated in terms of image content; 2) The training set and the test set account for 80% and 20% respectively Proportion; 3) When the rules 1 and 2 are met, the training set and the test set are randomly divided to evaluate the performance of the algorithm. This process is repeated 1000 times, and the median value of the results is taken as the final algorith...
Embodiment 2
[0102] In this implementation we use the entire LIVE database to train CPNR and run it on another popular image quality database TID2008 (see figure 2 ), and compare the results with two classical full-reference methods (see Table 5). It can be seen that although we are training on the LIVE database, the obtained CPNR also shows high subjective consistency in the TID2008 library, which is enough to prove that the method proposed in the present invention has a better ability to be independent of the database .
[0103] Table 5 SROCC comparison of the three methods in the TID2008 database
[0104]
PUM
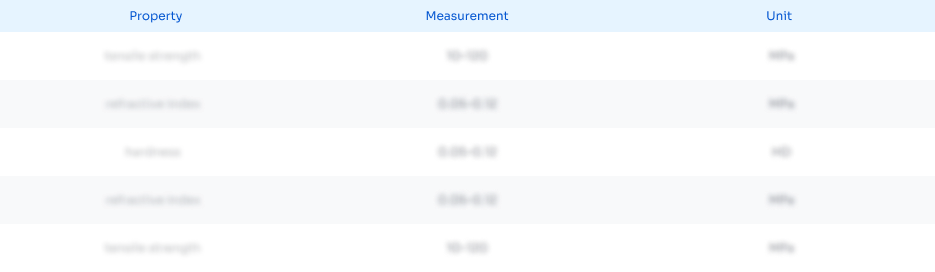
Abstract
Description
Claims
Application Information
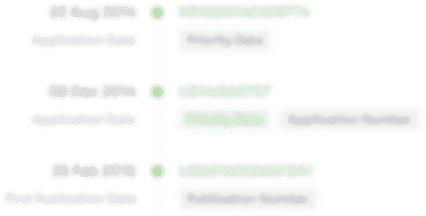
- R&D
- Intellectual Property
- Life Sciences
- Materials
- Tech Scout
- Unparalleled Data Quality
- Higher Quality Content
- 60% Fewer Hallucinations
Browse by: Latest US Patents, China's latest patents, Technical Efficacy Thesaurus, Application Domain, Technology Topic, Popular Technical Reports.
© 2025 PatSnap. All rights reserved.Legal|Privacy policy|Modern Slavery Act Transparency Statement|Sitemap|About US| Contact US: help@patsnap.com