Text feature extraction method based on categorical distribution probability
A technology of distribution probability and feature extraction, applied in special data processing applications, instruments, calculations, etc., can solve the problems of low classification accuracy, high word space dimension, and large amount of calculation, so as to improve processing efficiency, effect, and operation. effect of time reduction
- Summary
- Abstract
- Description
- Claims
- Application Information
AI Technical Summary
Problems solved by technology
Method used
Image
Examples
Embodiment
[0038] refer to figure 2 , the present invention needs to implement the effectiveness of the text feature extraction method of category distribution probability on a text classification task. By selecting a certain set of Chinese texts, the corpus texts are manually classified according to predefined categories. Perform preprocessing on the classified text set, and then perform feature extraction on the preprocessed text set to obtain a desired number of text feature word sets. The vector space is defined by the selected feature word set, and the preprocessed text is converted into the representation of the vector space model. The standard tfidf weight calculation method is adopted. Then use the specified classifier to train the text vector to obtain the trained classification model.
[0039] When it is necessary to classify the text to be classified, it is only necessary to convert the text to be classified into the representation of the vector space model on the feature ...
PUM
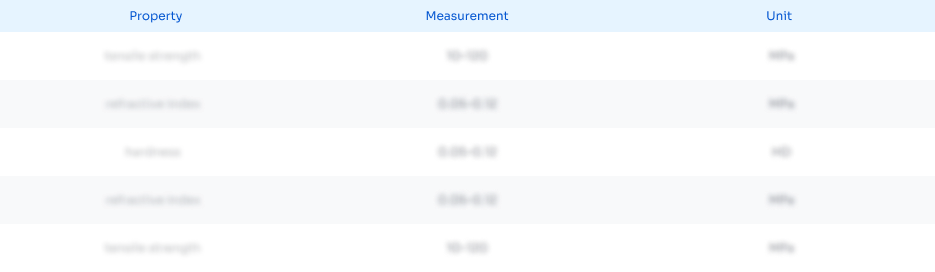
Abstract
Description
Claims
Application Information
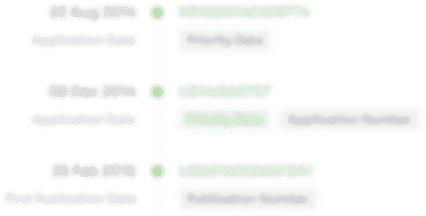
- R&D Engineer
- R&D Manager
- IP Professional
- Industry Leading Data Capabilities
- Powerful AI technology
- Patent DNA Extraction
Browse by: Latest US Patents, China's latest patents, Technical Efficacy Thesaurus, Application Domain, Technology Topic.
© 2024 PatSnap. All rights reserved.Legal|Privacy policy|Modern Slavery Act Transparency Statement|Sitemap