Method for selecting kernel function of support vector machine based on sample prior information and application
A support vector machine and prior information technology, applied in the field of support vector machine kernel function selection, can solve the problems of large randomness and limitations, different SVM promotion performance, lack of theoretical guidance, etc., to achieve fast operation speed and improve SVM learning The effect of ability and generalization ability
- Summary
- Abstract
- Description
- Claims
- Application Information
AI Technical Summary
Problems solved by technology
Method used
Examples
example 1
[0022] Example 1: Indian Liver Patient Dataset
[0023] Step A: The sample has a total of 579 data, with a dimension of 10. After data preprocessing, the O coordinate of the center of gravity of the hypersphere is calculated as (0.5058, 0.0000, 0.1073, 0.1786, 0.085, 0.3473, 0.1652, 0.4493, 0.5326, 0.4500), R=0.4984, α=0.9048.
[0024] Therefore, it is judged that the sample data has local distribution characteristics, and the radial basis kernel function with local characteristics is selected as the SVM kernel function type.
[0025] Step B: According to 80% of the known samples as the training set and 20% as the test set, 464 of the samples are used as the training set and 115 groups as the test set.
[0026] Step C: randomly select 3 sets of data:
[0027] ①The first training set is taken from number 1 # To 154 # , 194 # To 348 # , 388 # To 542 # , A total of 464 sample data. The test set was taken from the number 155 # To 193 # 349 # To 387 # 543 # To 579 # , A total of 115 sample...
example 2
[0034] Example 2: Balance Scale Data Set (Balance Scale Data Set)
[0035] Step A: The sample has a total of 625 data with a dimension of 4. After data preprocessing, the O coordinate of the center of gravity of the hypersphere is calculated as (0.6250, 0.5000, 0.2500, 0.7500), R=0.4507, α=0.2944.
[0036] Therefore, it is judged that the sample data has global distribution characteristics, and a polynomial kernel function with global characteristics is selected as the SVM kernel function type.
[0037] Step B: According to 80% of the known samples as the training set and 20% as the test set, 500 of the samples are used as the training set and 125 groups as the test set.
[0038] Step C: randomly select 3 sets of data:
[0039] ①The first training set is taken from number 1 # To 166 # 209 # To 375 # 418 # To 584 # , A total of 500 sample data. The test set was taken from the number 167 # To 208 # 376 # to # 417 # 585 # To 625 # , A total of 125 sample data.
[0040] ②The second set of ...
example 3
[0046] Example 3: Australian Credit Approval Data Set (Australian Credit Approval Data Set)
[0047] Step A: The sample has a total of 690 data with a dimension of 14. After data preprocessing, the O coordinate of the center of gravity of the hypersphere is calculated as (0.5000, 0.1479, 0.0105, 0.2500, 0.1923, 0.1875, 0.0007, 0.0000, 0.5000, 0.0299, 0.0000, 0.5000, 0.1050, 0.0050), R=0.8007, α= 0.9610.
[0048] Therefore, it is judged that the sample data has local distribution characteristics, and the radial basis kernel function with local characteristics is selected as the SVM kernel function type.
[0049] Step B: Take 80% of the total number of samples as the training set (ie 552 samples), and 20% as the test set (ie 138 samples).
[0050] Step C: randomly select 3 sets of data:
[0051] ①The first training set is taken from number 1 # To 184 # , 232 # To 416 # 464 # To 648 # , A total of 552 samples. The test set is taken from the number 185 # To 231 # 417 # To 463 # 649 # To ...
PUM
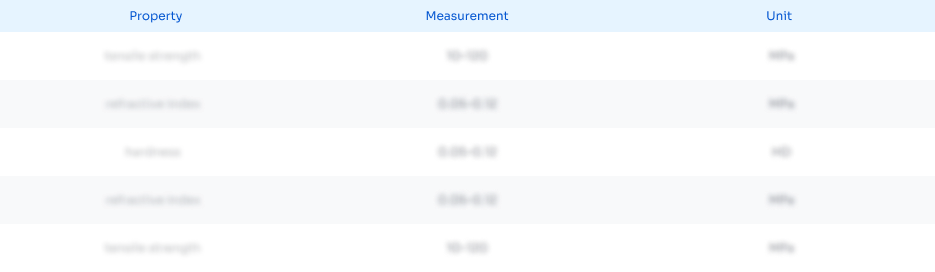
Abstract
Description
Claims
Application Information
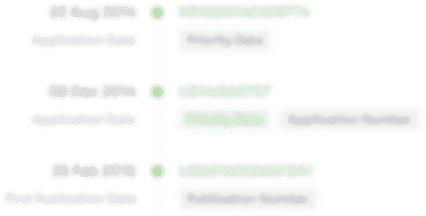
- R&D Engineer
- R&D Manager
- IP Professional
- Industry Leading Data Capabilities
- Powerful AI technology
- Patent DNA Extraction
Browse by: Latest US Patents, China's latest patents, Technical Efficacy Thesaurus, Application Domain, Technology Topic, Popular Technical Reports.
© 2024 PatSnap. All rights reserved.Legal|Privacy policy|Modern Slavery Act Transparency Statement|Sitemap|About US| Contact US: help@patsnap.com