Wavelet neural network weight initialization method based on Bayes estimation
A technology of wavelet neural network and Bayesian estimation, applied in the field of wavelet neural network optimization, can solve the problems of sample points affecting the probability density of weights and speed up the learning speed of wavelet network
- Summary
- Abstract
- Description
- Claims
- Application Information
AI Technical Summary
Problems solved by technology
Method used
Image
Examples
Embodiment Construction
[0058] The present invention will be further described below in conjunction with the accompanying drawings and embodiments.
[0059] Aiming at the research status and shortcomings of the existing wavelet neural network weight initialization technology, the present invention proposes a wavelet network that can effectively improve the learning efficiency and accuracy of the neural network in the initial stage, thereby accelerating the convergence speed and reducing the oscillation amplitude of the network output Weight initialization method. This method optimizes the learning of the wavelet network in the initial stage by integrating the wavelet type, network structure, input value and output index value into the weight state equation, thus providing a better data basis for the subsequent network learning and training.
[0060] The purpose of the present invention is to initially set the weight of the wavelet network based on Bayesian estimation, which uses the differential dyna...
PUM
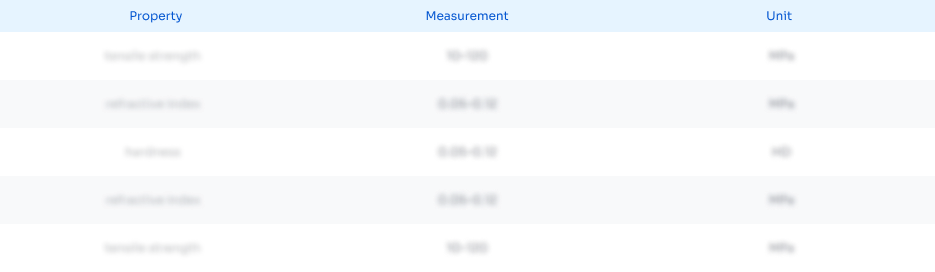
Abstract
Description
Claims
Application Information
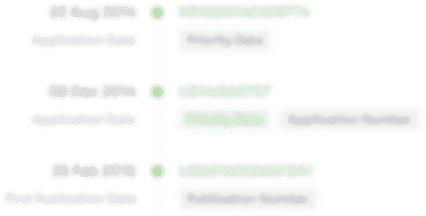
- R&D Engineer
- R&D Manager
- IP Professional
- Industry Leading Data Capabilities
- Powerful AI technology
- Patent DNA Extraction
Browse by: Latest US Patents, China's latest patents, Technical Efficacy Thesaurus, Application Domain, Technology Topic, Popular Technical Reports.
© 2024 PatSnap. All rights reserved.Legal|Privacy policy|Modern Slavery Act Transparency Statement|Sitemap|About US| Contact US: help@patsnap.com